Michael Griffiths
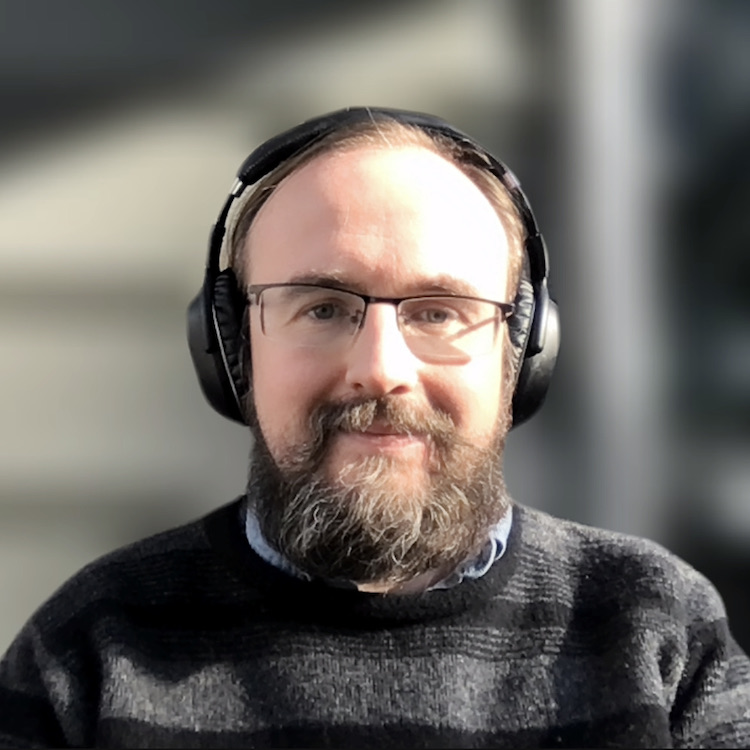
Michael Griffiths is a data scientist at ASAPP. He works to identify opportunities to improve the customer and agent experience. Prior to ASAPP, Michael spent time in advertising, ecommerce, and management consulting.
Generating New Customer Intelligence
Contact centers are goldmines of market information – from addressing customer issues and gauging their wants and needs, to seeing how they rate you in comparison to your competitors, and more. Customer interactions contain valuable information to improve current products and can provide early warning signals for any potential issues or emerging competition.
Despite the valuable information available, it has been incredibly difficult to access: deciphering phone audio or messaging transcripts is an arduous task. Text analysis has provided some assistance, but more often than not the optimal solution has been to ask the agent or customer on the call to fill out a survey with the data we care about.
Agents can be extremely effective at filling out these surveys, yet at a cost: adding questions is very expensive, and you are only able to acquire future data. Analyzing historical trends is still an onerous task. Requesting feedback from customers proves more difficult as well; sampling bias becomes an issue while other obstacles may occur. Leveraging quality management data for insights quickly runs into sparsity issues, making proactive responses too slow.
At ASAPP, we’ve incorporated Large Language Models (LLMs) to solve this problem for years as part of our Structured AutoSummary product. LLMs are great at understanding the meaning of the text. We can use them to regularize the recording of the interaction. We can represent conversations as a free text summary, and we can pull structured data out of conversations.
Newer LLMs can also perform a facsimile of reasoning. GPT4 and other models can be great at answering questions that require combining pieces of information in a call transcript. That extends the number of questions we can answer with high confidence – and the amount of structured data we can extract from conversations.
Structured data remains essential. Although LLMs can be very good at analyzing a single conversation, it takes a different approach to analyze hundreds or millions of customer interactions. Traditional analytics approaches – e.g. BI tools, Excel, ML models, etc – are the best way to analyze, identify patterns, and understand trends across a large amount of data. Now we can expose customer interaction data in a way those analytical tools understand.
Certainly, there are some complications in relying on AI to convert unstructured conversations to a usable structured format. At ASAPP, we’ve devoted substantial effort to managing hallucinations and reliable data collection by building in dedicated feedback loops and having multiple models working together that tackle different aspects of hallucinations.
Not surprisingly, the quality of data matters too. We’ve benchmarked the quality of our AutoSummary outputs against ASR accuracy (1-WER), and we see that highly accurate transcripts (where our own generative end-to-end ASR system AutoTranscribe sits in the mix) produce materially higher quality data on downstream tasks like extracting structured data out of conversations.
Turning unstructured audio and text into structured data unlocks a wealth of data stored in contact center records. Utilizing existing analysis tools and approaches can make contact center data available to other departments, like Research and Development, Marketing, and Finance, in real-time without purchasing additional IT capabilities for analysis and visualization.
For agents, this provides a massive boost in efficiency, getting quick answers to business questions and freeing up more time to help customers. For customers, it’s even more dramatic. They get answers faster and have a much smoother experience overall – all without survey bias.
LLMs are fantastic tools for language: writing poetry, essays, and code. They are also great at turning natural language into structured data, blurring or eliminating the boundary between “structured data” and “unstructured data.” Leveraging that data, and making it available to all the existing business processes, is where we’re heading with Structured AutoSummary.
How to Understand Different Levels of AI Systems
AI systems have additional considerations over traditional software. A key difference is in the maintenance cost. Most of the cost of an AI system happens after the code has been deployed. ML models degrade over time without ongoing investment in data and hyperparameter tuning.
The cost structure of AI systems are directly affected by these design decisions; the level of service, and improvement over time are categorically different across different levels. Knowing the level of the AI system can help practitioners and customers predict how the system will change over time – whether it will continuously improve, remain the same, or even degrade.
Levels of AI Systems start at traditional software (Level 0) and progress up to fully Intelligent software (Level 4). Systems at Level 4 essentially maintain and improve on their own – they require negligible work. At ASAPP we call Level 4 AI Native®.
Moving up a level has trade-offs for practitioners and customers. For example, moving from Level 1 to Level 2 reduces ongoing data requirements and customization work, but introduces a self-reinforcing bias problem that could cause the system to degrade over time. Choosing to move up a level requires practitioners to recognize the new challenges, and the actions to take in designing an AI system.
While there are significant benefits in scalability (and typically performance/robustness/etc) in moving up levels, it’s important to say that most systems are best designed at Level 0 or Level 1. These levels are the most predictable: performance should remain roughly stable over time, and there are obvious mechanisms to improve performance (e.g. for Level 1, add more annotated training data).
AI Levels
Designing AI systems is different from traditional software development, because the behavior of the system is learned – and can potentially change over time once deployed. When practitioners build AI systems, it can be useful to talk about their “level”, just like SAE has levels for self-driving cars.

Moving up a level has trade-offs for practitioners and customers. This requires practitioners to recognize the new challenges, and the actions to take in designing an AI system
Level 0: Deterministic
No required training data, no required testing data
Algorithms that involve no learning (e.g. adapting parameters to data) are at level zero.
The great benefit of level 0 (traditional algorithms in computer science) is that they are very reliable and, if you solve the problem, can be shown to be the optimal solution. If you can solve a problem at level 0 it’s hard to beat. In some respect, all algorithms–even sorting algorithms (like binary search) – are “adaptive” to the data. We do not generally consider sorting algorithms to be “learning”. Learning involves memory–the system changing how it behaves in the future, based on what it’s learned in the past.
However, some problems defy a pre-specified algorithmic solution. The downside is that for problems that defy human understanding (either once, or in number) it can be difficult to perform well (e.g. speech to text, translation, image recognition, utterance suggestion, etc.).
Examples:
- Luhn Algorithm for credit card validation
- Regex-based systems (e.g. simple redaction systems for credit card numbers).
- Information retrieval algorithms like TFIDF retrieval or BM25.
- Dictionary-based spell correction.
Note: In some cases, there can be a small number of parameters to tune. For example, ElasticSearch provides the ability to modify BM25 parameters. We can regard these as tuning parameters, i.e. set and forget. This is a blurry line.
Level 1: Learned
Static training data, static testing data
Systems where you train the model in an offline setting and deploy to production with “frozen” weights. There may be an updating cadence to the model (e.g. adding more annotated data), but the environment the model operates in does not affect the model.
The benefit of level 1 is that you can learn and deploy any function at the modest cost of some training data. This is a great place to experiment with different types of solutions. And, for problems with common elements (e.g. speech recognition) you can benefit from diminishing marginal costs.
The downside is that customization to a single use case is linear in their number: you need to curate training data for each use case. And that can change over time, so you need to continuously add annotations to preserve performance. This cost can be hard to bear.
Examples:
- Custom text classification models
- Speech to text (acoustic model)
Level 2: Self-learning
Dynamic + static training data, static testing data
Systems that use training data generated from the system for the model to improve. In some cases, the data generation is independent of the model (so we expect increasing model performance over time as more data is added); in other cases, the model intervening can reinforce model biases and performance can get worse over time. To eliminate the chance of reinforcing biases, practitioners need to evaluate new models on static (potentially annotated) data sets.
Level 2 is great because performance seems to improve over time for free. The downside is that, left unattended, the system can get worse – it may not be consistent in getting better with more data. The other limitation is that some systems at level two might have limited capacity to improve as they essentially feed on themselves (generating their own training data); addressing this bias can be challenging.
Examples:
- Naive spam filters
- Common speech to text models (language model)
Level 3: Autonomous (or self-correcting)
Dynamic training data, dynamic test data
Systems that both alter human behavior (e.g. recommend an action and let the user opt-in) and learn directly from that behavior, including how the systems’ choice changes the user behavior. Moving from Level 2 to 3 potentially represents a big increase in system reliability and total achievable performance.
Level 3 is great because it can consistently get better over time. However, it is more complex: it might require truly staggering amounts of data, or a very carefully designed setup, to do better than simpler systems; its ability to adapt to the environment also makes it very hard to debug. It is also possible to have truly catastrophic feedback loops. For example, a human corrects an email spam filter – however, because the human can only ever correct misclassifications that the system made, it learns that all its predictions are wrong and inverts its own predictions.
Level 4: Intelligent (or globally optimizing)
Dynamic training data, dynamic test data, dynamic goal
Systems that both dynamically interact with an environment and globally optimizes (e.g. towards some set of downstream objectives), e.g. facilitating an agent while optimizing for AHT and CSAT, or optimizing directly for profit. For example, an AutoCompose system that optimizes for the best series of clicks to optimize the conversation.
Level 4 can be very attractive. However, it is not always obvious how to get there, and unless carefully designed, these systems can optimize towards degenerate solutions. Aiming them at the right problem, shaping the reward, and auditing its behavior are large and non-trivial tasks.
Why consider levels?
Designing and building AI systems is difficult. A core part of that difficulty is understanding how they change over time (or don’t change!): how the performance, and maintenance cost, of the system will develop.
In general, there is increasing value as you move up levels, e.g. one goal might be to move a system operating at Level 1 to be at Level 2 – but complexity (and cost) of system build also increases as levels go up. It can make a lot of sense to start with a novel feature at a “low” level, where the system behavior is well understood, and progressively increase the level – as understanding the failure cases of the system becomes more difficult as the level increases.
The focus should be on learning about the problem and the solution space. Lower levels are more consistent and can be much better avenues to explore possible solutions than higher levels, whose cost and variability in performance can be large hindrances.
This set of levels provides some core breakpoints for how different AI systems can behave. Employing these levels – and making trade-offs between levels – can help provide a shorthand for differences post-deployment.
Matrix Layout
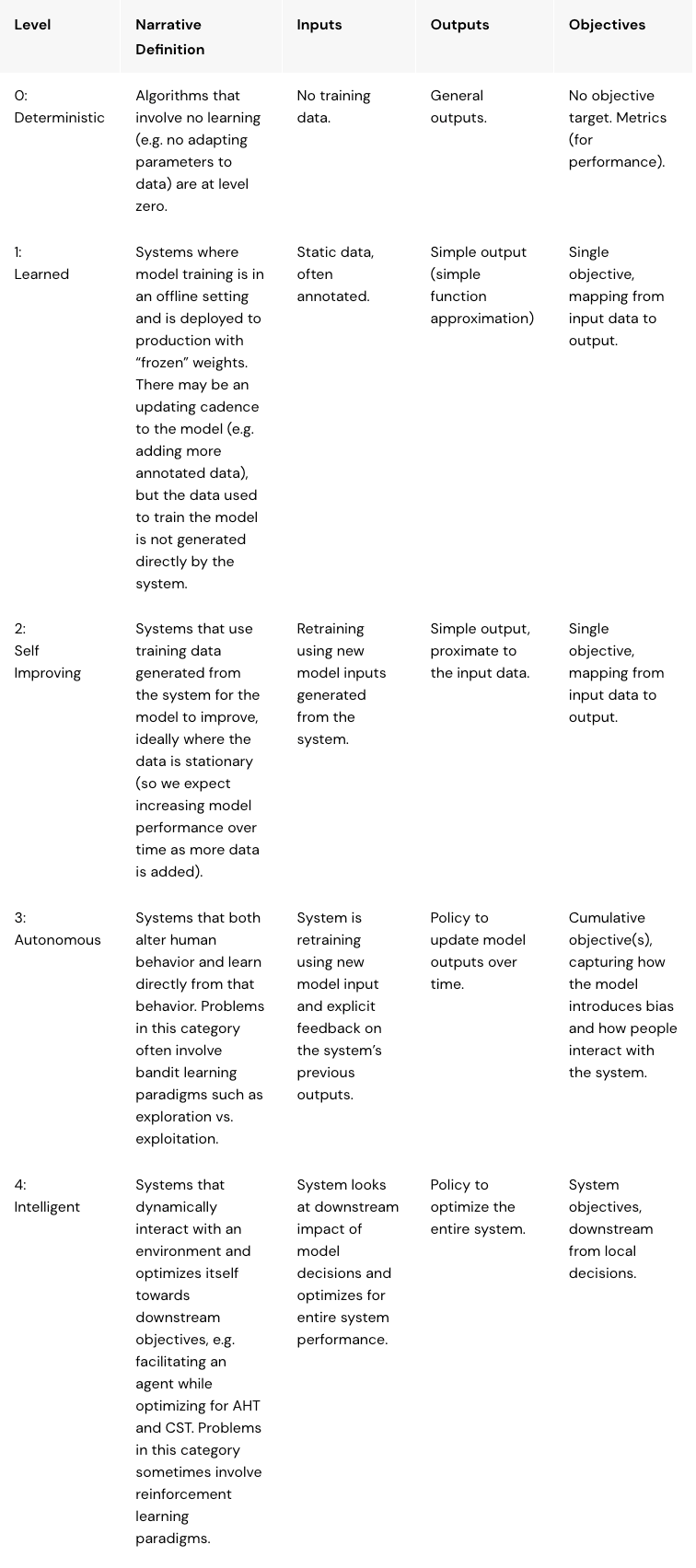
How do you find automation workflows for your contact center?
Automating common tasks and enabling self-service issue resolution for customers is an essential part of any online customer service experience. These automated flows directly address a specific well-scoped problem for the customer, getting them to resolution quicker and freeing up agents to handle more complex issues. But, automation doesn’t have to be an all or nothing proposition. At ASAPP, we automate flows before, during, and after agent interactions, increasingly reducing agent workload and growing the opportunity for self service over time.
Discovering and prioritizing new flows and understanding what’s needed for successful automation, however, can be challenging. It is often a time consuming and labor intensive process. ASAPP has developed AI Native® approaches to surface these workflows to humans, and we’ve been awarded a patent, “Identifying Representative Conversations Using a State Model” for a powerful solution we developed to perform flow induction.
It’s difficult for a human to imagine all the possible conversation patterns that could be automated, and which ones are most important to automate. It’s important to consider things like how many users it would affect, how much agent time is being spent on the intent, whether the flow has a few well-defined paths or patterns, what value the intent brings to the business, and whether there are any overlaps between this intent and other conversations.

Rather than manually sifting through all the data, an analyst can leverage patterns identified by the model to more quickly deploy automated workflows and evaluate their potential with real usage data.
Michael Griffiths
We call the process of automatically discovering and distilling the conversational patterns—“workflows”, or “flows” for short—flow induction. We can condense a large collection of possible flows to a much smaller number of representative flows. These induced flows best capture interactions between customers and agents, and flags where automation can lend a helping hand. This facilitates faster and more comprehensive creation of automated flows, saving time and money.
Our patented approach for flow induction begins by representing each part of a conversation mathematically, capturing its state at the time. As a simple example, we would want the start of each conversation—where agents say “hello” or “how are you” or “welcome to X company”—to be similar, with approximately the same state representation. We can then trace the path the conversation traces as it progresses from start to finish. If the state is two dimensional, you could draw the line that each conversation takes as its own “journey.” We then group similar paths and identify recurring patterns within and across conversations.
The process of identifying automation use cases is dramatically simplified with this representation. Instead of manually sifting through conversations, talking to experienced agents, or listening into calls to do journey mapping—the analyst can dive into a pattern the model has identified and review its suitability for automation. Even better, because ASAPP is analyzing every customer interaction, we know how many customers are affected by the flows and what the outcomes (callback, sales conversion, etc) are — making prioritization a breeze.
ASAPP deploys “flows” like this across our platform. By identifying the recurring work that agents are handling an analyst can construct integrated flows for agents to serve in any part of a conversation. And over time, more and more flows can be sent directly to the customer so they can self-serve. Once deployed every flow becomes part of a virtuous feedback loop, where usage informs how impactful the automation is for our customers and their customers. This process informs both new flow opportunities and refinements to existing flows.
The chatbot backlash
Chatbots got a bad name because they’ve been overused. The tech isn’t appropriate for complex issues.
Contact centers are under tremendous pressure: they need to solve a growing array of increasingly complex customer problems while under mounting pressure to reduce costs.
Companies have adopted technologies—like chatbots and IVRs—that “deflect” customers from agents. This helps to address volume and cost challenges; it does not help with increasing complexity, nor with the growing number of problems customers need help with. Companies that implement chatbots or IVRs may focus on “deflection” or containment as a measure of success; this is a flawed strategy that distracts from overarching customer service goals.
The ability of chatbots and IVRs to solve customer problems has been oversold in the last decade. Even the highest performing systems are designed around inflexible rule-based models. These technologies are not new. An industry standard for chatbots was finalized in 2001, and the industry standard for IVRs was finalized a year earlier; indeed, the underlying technology is very similar. The advent of neural natural-language-processing (NLP) models in the early 2010s led to a resurgence in chatbot interest. While performance has improved (for example, you can classify a customer problem in one step, instead of forcing a customer to navigate a menu), the systems remain rule-based and worse, fragile.
The fragility of chatbots means constant tuning and re-working of the rules. It’s simply a budget shift from agents to IT departments or external consultants.
The fundamental limitation of chatbots
Chatbots and IVRs are extremely useful technologies when used appropriately. However, they are not a panacea—and attempting to use these technologies for all customer problems gives them a bad name. They’ve been sent in to do the wrong job—of course they are going to fail to impress.
Rule-based systems (like chatbots and IVRs) can handle simple problems, and improve discoverability of self-service options. This is a good thing. Customers can rely upon a single entry point for support, and be gently routed to the best way to solve their problem. In many cases, that can be showing the customer how to get what they need from digital self-service; in others, the customers’ problems can be addressed with automated responses within the chat interface. Customers are happy because their problems are solved faster (and with no hold time!); and companies are happy because it reduces total cost of service.
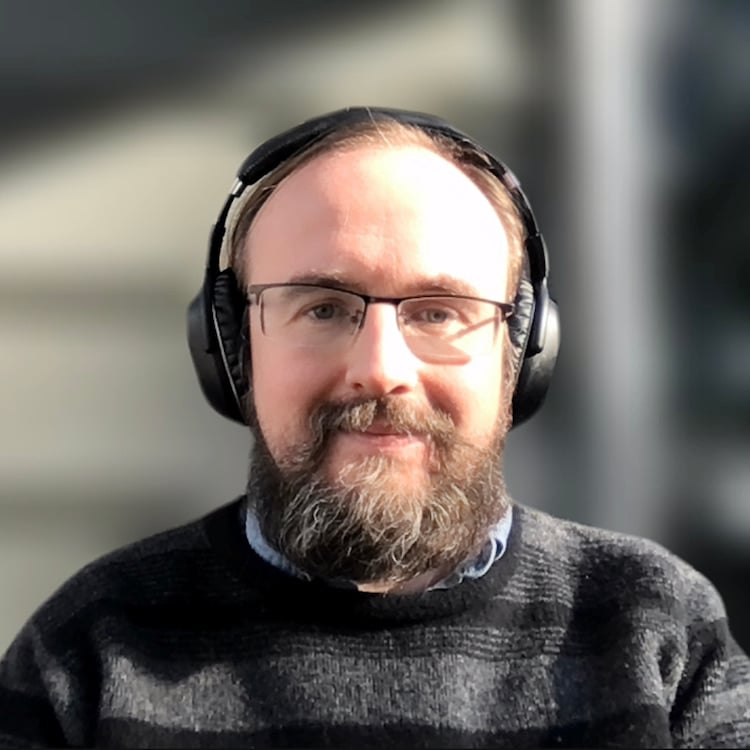
Chatbots don’t serve companies well as a siloed tool focused purely on deflection. But, this type of automation can help customers find self-serve answers to simpler needs. Plus, it can help the agent—attempting first troubleshooting steps before escalating to the agent with full context, for example.
Michael Griffiths
The idea is to streamline the customers’ path to resolution—without deflecting customers who will be best served by an agent. Siloed support channels and a focus purely on deflection can result in both a fragmented customer experience and duplication of effort and investment by the company (for example: creating help content in web self-service applications as well as programming it into a chatbot, for example.) Educating customers to use existing self-service tools, where appropriate, can minimize that duplication—as well as streamline and improve the customer experience. Likewise, it’s important to provide a easy path from bot to live-agent interaction when a customer need can’t be addressed by the bot—to avoid making customers hop from channel to channel, starting their journey over with each one.
Chatbots are an excellent way to help shift incoming customer contacts from messaging, voice, or the web into digital self-service when appropriate. But, they cannot serve as the end goal—and using chatbots won’t close down your contact center. The scope of the problems that chatbots can handle is limited. It is perfectly reasonable for these systems to address upwards of 30% of customer issues, though they are often the easy issues and so the reduction on total agent time spent is less than that. To transform customer experience, companies must also look for meaningful ways to help those agents.
Resolving the increasing pressure on agents
As self-service and automation through IVRs and chatbots address more and more simple customer needs, the conversations that do get to agents are increasingly difficult. The result: Agents are handling more complex problems, handle times are longer, and cost savings never materialize.
What does it take to realize those promised cost savings? Our approach is to bring artificial intelligence right to the agent. We realized that the more you invest in customer self-service and engage chatbots for the simpler interactions, the more you need to help your agents handle parts of the job inside the conversation.
The benefits of pairing humans with AI are dramatic. We unite the best of human agents with the best of machine intelligence: more than that, we solve the problem of chatbot management.
Agents use artificial intelligence to make their job easier,It automates portions of their workflow. The AI monitors what agents use, what the outcome is, and what is truly effective for improving the customer experience—and improves itself over time. This feedback cycle means that as the system learns, agents use it more (e.g. from 15% of the time to 60% of the time a year later); and it means that the system learns from your best agents to help move every interaction in the right direction.
The best part of this approach is that we keep the benefits of chatbots. AI-driven automation trained by your agents can replace rule-based chatbots—and be used throughout the conversation, including before the customer is connected to the agent.
The Future of Work
Enterprises would be well served to determine how to eliminate significant minutes of work. By bringing AI to the agent—where upwards of 80% of CX budgets are spent today—provides a dramatic increase in value. Our systems learns how best to help those agents. It is the union of human and AI, and the development of a more robust system, that will transform the contact center.