Finding the Path Forward Between Hype and Hesitation
In the midst of the well-deserved hype around generative AI for customer service, there are reasons for caution.
We’ve all heard the horror stories about early AI bots in the wild: Bots that encouraged people to break laws or do unsafe things. Bots that agreed to sell big-ticket items for pennies on the dollar. Bots that spouted offensive and biased language or disparaged the brand they supposedly represent. It seems that some bots can be convinced to break the rules.
Meanwhile, customers are growing increasingly concerned about data security and wary of how their personal data is used to train large language models (LLMs). And the future of the regulatory landscape grows murkier by the day as governments grapple with the implications of rapid technological innovation.
It can all feel like being stuck between a rock and a hard place, making it difficult to know how – or even if – you should move forward with customer-facing generative AI solutions.
Generative AI is still a new technology for contact centers. If that newness gives you pause, good. A dose of healthy skepticism is useful for managing risk.
But as with other technologies, the early adopters gain a competitive advantage. And the early adopters have already tested the waters with initial deployments of generative AI in customer-facing roles. Now, they’re realizing substantial value with AI agents that handle customer interactions on their own – safely, efficiently, and in a way that customers appreciate.
These success stories aren’t rare, and they don’t require extraordinary luck.
Success with generative AI agents depends on a solid understanding of the risks and a clear plan to mitigate and manage them. That’s the approach the early adopters are taking.
With the right information, you can do it, too. Safely.
Security vs. safety: Understanding the distinction
The words security and safety are sometimes used interchangeably when talking about generative AI. And they are related and complementary. But understanding the distinction between AI security and AI safety is crucial for developing a comprehensive strategy for trust with generative AI solutions.
AI Security
AI security primarily focuses on safeguarding against external threats, such as malicious attacks and data breaches. A good example is prompt injection, in which a bad actor attempts to disguise malicious input as a legitimate prompt to manipulate the AI into leaking sensitive data, granting access to restricted systems, or saying something it shouldn’t.
Much of the work of maintaining security with a generative AI solution rests on foundational security controls that are not specific to the AI, such as data protection, access controls, monitoring and logging, and incident response plans.
AI Safety
AI safety focuses on preventing unintended harm and other negative consequences that could arise from how the generative AI solution performs. The goal is to ensure that the solution stays on task, produces accurate information, and operates ethically. For example, some safety measures focus on preventing the AI from making offensive statements or misleading people with inaccurate information.
To ensure safety, generative AI solutions must include specific safety mechanisms as guardrails, robust quality assurance procedures that account for the open-ended nature of generative AI, and a clear process for human oversight of the AI agent.
How Security and Safety Intersect
While security and safety address different aspects of generative AI, they often intersect and influence each other. For instance, a breach in AI security could lead to safety issues if malicious actors manipulate the AI to produce harmful content. Similarly, lapses in AI safety mechanisms could make the system more vulnerable to security threats. A balanced approach that addresses both security and safety is essential for developing and deploying a trustworthy generative AI solution.
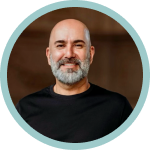
It’s important to differentiate between these two terms that often get commingled.
- Khash Kiani, Head of Security and Trust, ASAPP
What causes generative AI to make mistakes?
To err is human. It’s also AI. Or it can be under certain conditions. And for better or worse, large language models (LLMs) are trained to mimic humans.
With traditional programming and deterministic bot flows, errors occur as a result of programming. But with generative AI, the errors are less predictable, less consistent and harder to source. There are a few key reasons why generative AI makes these mistakes.
The inherent nature of generative AI
Traditional automated systems, like IVRs and chatbots, are consistent. They produce the same results every time. But that consistency comes at a cost. They do only what they were specifically programmed to do by humans who configured the various options and flows. That severely limits their application and makes them difficult and costly to build and maintain. It also means that their errors are directly caused by bad programming choices.
Generative AI is different. Because it creates new content based on inputs and context, the possible outputs are unlimited.
This open-ended nature of generative AI creates both new benefits and new risks. In that respect, it behaves a bit like human agents.
No one model is right all the time
There’s an adage about human nature that says the collective wisdom of a group tends to outweigh what any single person contributes. This dynamic holds true for generative AI models also. No single model excels at everything. Each one is built with different parameters for different objectives. Some are designed for a wide range of applications, while others have a narrower purpose. Some emphasize quality over speed and cost, and some are designed to be faster or more cost-effective, even if it means they're less accurate.
The work of an AI agent can be quite varied, even when the agent is tasked with a narrow set of objectives. Handling an interaction that requires modifying a user’s account can require many steps and many actions with different underlying components. No single model will excel at all of them. When AI solutions rely too heavily on individual models, their performance, security, and speed can suffer.
Ungrounded models rely on their intrinsic knowledge
Generative AI depends on Large Language Models (LLMs), which offer two things: general knowledge about the world and the ability to reason. To acquire these skills, LLMs need to be trained on a large body of content. That means they contain out-of-domain data, or in other words, information that is not relevant to your business.
It’s also important to note that this training represents a moment in time. And as time passes, some of the information included becomes outdated and even inaccurate. So, even if the training data included information about your business, that information might no longer be true.
As a starting point, that’s not very different from a new hire who’s never worked as a customer service agent before and doesn’t know much (or anything) about your business or industry. Your new hire might have amazing communication skills and a wide range of general knowledge about basic topics. But they still need to be grounded in up-to-date information specific to your business, such as product details, internal policies, and preferred terminology to represent your brand the way you expect.
An AI agent has similar needs. The LLMs it relies on acquired their skills from a broad base of data that has nothing to do with your business. To improve accuracy, it must be grounded in your policies, processes, and other information specific to your business. Generative AI agents that are not sufficiently grounded will fall back on their intrinsic knowledge, which was acquired with the initial training of the LLM. That increases the likelihood of mistakes.
Without guardrails, AI can be exploited
Bad actors will exploit any vulnerability they find. Sometimes the vulnerability is human, sometimes it’s technology. Humans can be tricked into revealing sensitive information. And they can be convinced by others or even their own objectives to spy on people within the company, expose confidential information, or use company time for personal gain.
Without guardrails in place, AI can be exploited, too. Naive deployments can lead to a range of problems. For instance, a customer service chatbot could be misused to retrieve data the user should not be able to access. Or it could be convinced to spout offensive language, disparage its own company, recommend a competitor instead, or even agree to sell a pricey product for a pittance.
Whether human or AI, an exploited agent can damage a brand’s reputation and compromise security and safety for both the company and its customers.
All mistakes aren’t the same: Hallucinations and other inaccurate output
The causes of inaccurate output from generative AI are varied. The types of mistakes are varied, too.
When AI produces inaccurate responses, we often hear them labeled as hallucinations. But not all errors are hallucinations. And not all hallucinations are equally bad. It’s tempting to think that the distinctions don’t really matter. After all, wrong is wrong. But treating all undesirable outputs as equally bad can make it difficult to identify root causes and improve performance.
To understand the kinds of errors generative AI can make, consider two characteristics:
- Validity: Is the response true information or misinformation?
- Justification: Did the response come from a company-specified source, such as the knowledge base (justified) or from the model’s intrinsic knowledge (unjustified)?
When the output is valid (true information), the customer sees no mistake, regardless of whether it came from a trusted source (justified) or not. When the output is not valid (misinformation), the AI agent could mislead the customer, regardless of whether the output was based on a trusted source (justified).
These combinations of validity and justification create a simple grid of outputs, each with its own level of risk and possibilities for mitigation and prevention.
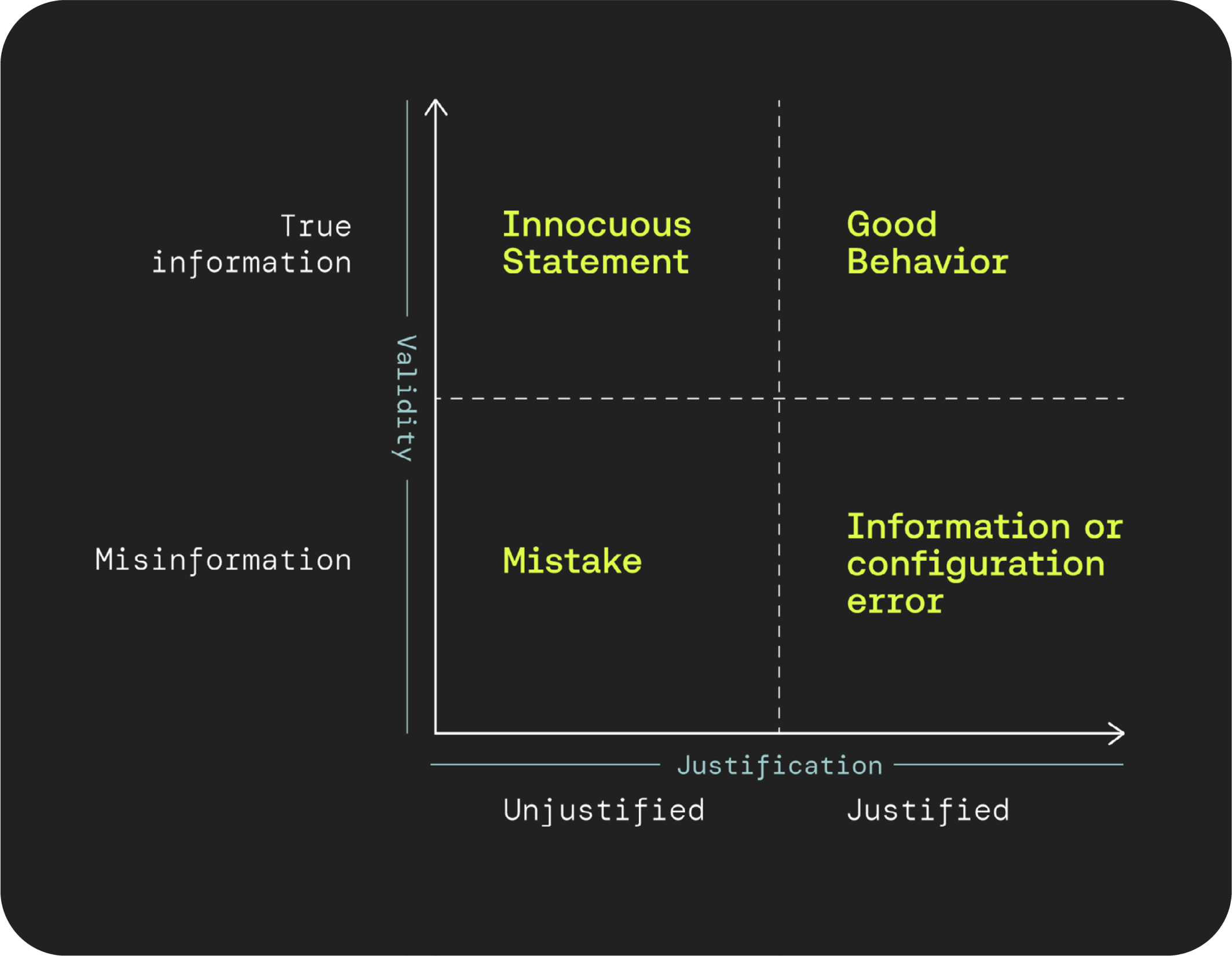
Good behavior: True information and justified
When the output of the AI agent is true and it’s based on a trusted source, the result is exactly what we’re looking for.
Information or configuration error: Misinformation and justified
This type of error occurs when the output of the AI agent is inaccurate but is based on a trusted source. For example, after consulting the knowledge base, an AI agent might inform the customer that there is no fee for returning a previously purchased product. If the return policy actually requires a restocking fee but the knowledge base says otherwise, the AI will provide inaccurate information. This kind of error could be apparent to the customer and create frustration. But it is easy to resolve. Once the knowledge base is updated, the AI will provide the correct information in future interactions.
Innocuous statement: True information and unjustified
When the output of the AI agent is true but it’s not based on a trusted source, the customer does not perceive a problem. For example, an AI agent might tell an airline customer that boarding typically begins 30-40 minutes before the scheduled departure time. If that information did not come from a trusted source designated by your organization, then the statement is unjustified. Because the information is true, though, it does not pose a threat to your brand’s credibility. It is, however, a hallucination because the AI generated the output from its intrinsic knowledge.
Mistake: Misinformation and unjustified
This kind of error is the most concerning. The AI has generated inaccurate information (hallucination) and has misled the customer. To prevent this type of error from misleading customers, you will need some safety mechanisms in place, including technology guardrails built into your AI solution. You will also need to work with your AI solution provider to ensure that the instructions provided to the AI agent are sufficiently clear to reduce the likelihood of errors. Even with these measures in place, it’s not possible to eliminate errors completely. In that respect, generative AI is similar to your human agents. With that in mind, it’s important to develop quality systems to detect and handle errors when they do occur.
Much of the news coverage of the risks associated with AI fixates on hallucinations and bad actors jailbreaking systems. That fixation both exaggerates the problem and glosses over the nuanced set of challenges in deploying generative AI agents, which puts contact centers at a disadvantage. If you don’t know what kinds of errors can occur and what causes them, you can’t take meaningful steps to prevent mistakes or mitigate risks. Not all mistakes are hallucinations, and not all hallucinations are equally problematic. Some mistakes are caused by inaccuracies in the systems the AI relies on. And that’s something you have control over. While hallucinations cannot be completely eliminated, their occurrence can be dramatically reduced. And when they do happen, they can be caught and managed effectively.

By focusing on grounding responses in reliable data, employing safety mechanisms, and fostering continuous improvement, we can ensure that AI responses are safely grounded and free from harmful hallucinations.
- Heather Reed, Product Manager, ASAPP
Chatbots vs. generative AI agents: Comparing apples and oranges
Because a generative AI agent is a technology solution, the most obvious comparison is to traditional chatbots, many of which are powered by traditional forms of AI to help them understand intent and classify text. But traditional bots are built on deterministic conversation flows and are not capable of generating new content or autonomously taking action. Their outputs are more limited. And that’s where the comparison breaks down.
Because generative AI is more capable and completely open-ended, it is more helpful to consider how generative AI agents stack up against human agents in terms of overall performance and potential for errors. Humans are still capable of handling more complex customer issues and conversations than generative AI agents – at least for now. But they are costly to hire, train, and onboard. They’re also susceptible to a wider range of mistakes and undesirable behaviors than AI is, especially if the AI solution provider prioritized safety throughout design and development.
Consider these common issues with contact center agents and which ones are possibilities for a generative AI agent designed with robust safety mechanisms.
Generative AI agents are not perfect. Neither are humans. They both occasionally make mistakes. But just as imperfect humans can be managed to perform well in customer-facing roles, so can AI agents.
With the right oversight, generative AI agents built with robust safety mechanisms create no more risk than humans.
Leveraging existing quality management systems to improve safety with generative AI agents
Your organization might be new to deploying AI-powered capabilities, and that can generate some uncertainties about how you will manage AI and its associated risks over time. But within the contact center, you very likely have more tools and expertise at your disposal than you realize.
The strategies and processes you currently use for quality and performance management will be especially helpful. Many of them can be adapted to help you manage your AI agents in much the same way that you manage human agents.
Onboarding
When you hire new agents, they start with an onboarding program that teaches them about your products, processes, and expectations for the customer experience. And you grant them access to the systems they will need to access to resolve customers’ issues. After that initial onboarding, your agents probably receive training at regular intervals to reinforce their learning.
Your AI agent will need onboarding, too. They will need to be trained on your processes and granted access through APIs to relevant sources of information, such as your CRM and knowledge base. Your solution provider should work with you to establish a period for this onboarding, along with procedures for evaluating and optimizing the solution’s performance. The good news is that AI does not forget things the way humans do, so you won’t need to provide regular refresher training.
Performance monitoring
Your quality management function is already collecting performance data and analyzing it to inform improvement efforts. This effort might uncover patterns in key metrics across your teams of agents, like significant differences in Average Handle Time (AHT) or First Contact Resolution (FCR). Or they might identify trends that demonstrate improving or eroding efficiency for certain queues or types of customer issues.
These efforts will be just as useful when applied to your AI agents. A low FCR or customer satisfaction metric points to a problem that you can troubleshoot to improve both efficiency and the customer experience your AI agent is delivering. When it comes to performance monitoring, your AI agent is just another member of your frontline contact center team.
Data-driven coaching and optimization
An essential part of managing human agents is coaching them into better performance over time. The availability of real-time performance data in contact centers has opened up new opportunities for timely coaching that targets agents’ development needs.
The same kind of performance data can also be used to drive improvement with your AI models. For example, let’s say your AI agent is escalating more interactions to a human than you’d like. When you dig into the data, you might find that it needs information that doesn’t exist in your knowledge base. Or maybe it needs data from a system it’s unable to access. In either case, the cause is clear, and so is the solution. By monitoring performance and identifying specific weaknesses, you can improve the effectiveness of an AI agent with targeted interventions.
Compliance management
No matter how good the customer experience is with an AI agent, the costs will outweigh the benefits if it creates compliance issues. Fortunately, your quality management system already designs procedures to monitor and ensure compliance for human agents. Your AI agents can also rely on these procedures.
For both human and AI agents, call recording, full transcripts regardless of channel, and interaction summaries with structured data provide necessary documentation you can mine for quality and compliance.
Additional steps you can take to manage risk with AI agents
Any AI solution provider should offer both technology controls and guidance on steps you can take to deploy AI agents safely. Mitigating and managing risks with AI should be a shared responsibility between your organization and your AI solution provider.
The steps you normally take to ensure safety with a traditional technology are just as important with generative AI. But there are some additional considerations, too. As you prepare to onboard AI agents, these strategies should guide your approach to managing risk.
Start small and expand your use cases at your own pace
A large part of the appeal of generative AI is that it can “do anything.” But an open-ended mandate becomes dangerous quickly when you deploy it in a customer-facing role. It’s all but impossible to successfully configure, test, and optimize the system across all use cases at once.
It’s safer to start with narrow use cases that will limit the job function of the AI agent. A narrow, well-defined role offers natural safeguards and opportunities for your solution provider to help you put additional guardrails in place to improve safety and optimize performance.
For example, instead of deploying a generative agent to handle any kind of Tier 1 interaction, you might choose to start by having it handle only certain kinds of customer account modifications that can be addressed through a consistent sequence of steps.
This sequence does not need to be simplistic or deterministic. It might require the AI agent to assess the customer’s specific needs, look up their current account, determine which modifications are possible, request input from the customer, draw information from the knowledge base or other internal systems, perform the actions required to modify the account, and inform the customer of any implications of the modification, such as a change in price.
Within this narrow use case, you can maintain high expectations for performance and accuracy. In other words, narrow your expectations at the outset, but don’t lower them. Then, you can plan to expand to additional use cases at your own pace.

Clean up your data
The garbage in/garbage out rule is just as relevant with AI as it is with other kinds of technology, so it’s important to clean up the data sources your AI will rely on, like your CRM, knowledge base, and other systems of record. Accuracy is crucial. But you’ll also want to ensure that these systems use the right brand language so the AI agents use your preferred terminology.
If you’re deploying an AI agent on a voice channel, you’ll want to make sure that your transcription solution delivers exceptional accuracy with low latency. Inaccurate transcripts will degrade the performance of your AI agent. And delays in transcription create delays in service that frustrate customers, making them less satisfied with the experience your AI agents deliver.
Keep in mind that your data does not need to be perfect to get started with AI agents. But the more accurate it is, the better your agents will perform. That holds true for both human and AI agents. By starting with a narrow job function for your AI agent, you can focus your data cleanup to align with your targeted use cases and avoid a more substantial upfront overhaul of your knowledge base and other relevant systems. Then, as you expand to additional use cases, you can clean up more data and optimize knowledge as you go.
Plan to provide ongoing oversight
Deploying AI agents isn’t a set-it-and-forget-it operation. Just as your employees require regular supervision, coaching and redirection, your AI agents need ongoing oversight, too. Be prepared to work with your vendor to monitor the initial results during a predefined test period and make adjustments as needed. And plan to continue providing oversight continuously.
Conditions can change over time, such as shifts in customer behavior, updates to your company’s products, policies and services, and adjustments to business priorities. You’ll want to ensure that relevant systems and knowledge articles reflect these updates. You’ll also need to test that your generative AI agent is using the updated information appropriately.
Keep a human in the loop
Generative AI agents are more effective – and safer – when they have a human coworker who can step in when needed. When the AI agent hits a roadblock, like lack of access to the systems required to resolve the customer’s issue, it needs a human to assist. And when a conversation crosses into subject matter that requires a more nuanced approach, the human agent can take over.
You could also decide that, as a matter of policy, some types of interactions will always require a human in the loop. For example, you might choose not to allow an AI agent to perform certain tasks without direct authorization, such as approving refunds, modifying accounts, or offering non-standard discounts. In these cases, the AI agent will need a human partner who can authorize those actions.
Having a human in the loop also creates a safety net during your initial deployment of a generative AI agent. That builds confidence as you transition some types of interactions from humans to AI.
You’ll want to consider the kinds of interactions your AI agent will handle and the expertise that will likely be needed as you identify good candidates to serve as the human in the loop. Depending on the specific use cases your AI agent handles, the expert who works with it could be a Tier 2 support agent, senior CSM, quality assurance analyst, or other subject matter expert.
How your AI solution provider should ensure safety
Regardless of how much you do to mitigate risks and lay the groundwork for AI safety in your own organization, one question remains: How well does the solution itself reduce risk? It’s a question you should ask any AI vendor you’re considering.
At a minimum, your solution provider should be able to explain how they are implementing these strategies and measures.
A compound system that incorporates multiple LLMs
As capable as LLMs are, these monolithic models are not driving the state-of-the-art results that industry leaders are seeing. Compound systems are.
A compound system uses interacting components, including multiple models, data retrieval methods and other tools. At the center is an engine that orchestrates the models, using each one for what it does best. Maintaining a narrow scope for each model’s use enables greater precision. There is no single model that outperforms all others for every task. Each one is tuned to its own set of performance goals. A compound system takes advantage of these differences to improve accuracy and efficiency.
Because of its open-ended nature, generative AI models can’t be completely controlled. It’s impossible to guarantee that any single LLM will avoid mistakes or undesirable behaviors. But a compound system that incorporates models purpose-built for safety is much more capable of detecting and preventing unsafe behavior.
Such a system also has other components with real-time access to accurate and up-to-date information, such as customer data and your business policies. By grounding the system in this company-specific information, the AI agent can take advantage of the reasoning capabilities of the various models it uses without relying on their intrinsic knowledge.
To fully understand the benefits of a compound system, it can be helpful to consider the steps a human agent takes when solving a customer’s problem. Without thinking about it, the agent first assesses whether the customer is behaving in good faith. The agent listens to the customer to understand their problem, and then follows a series of steps to resolve it. A generative AI agent should follow a similar approach – with components that detect unsafe input, a structured process to understand and resolve a customer’s problem, and the ability to access a knowledge base or a transactional system to help the customer. A successful compound system creates a specific yet flexible workflow, grounded on company-specific information.
Input and output safety mechanisms
The solution should be able to detect when someone is trying to exploit the system by asking it to do something it should not. It should include mechanisms that detect and block malicious inputs, including code that would effectively redirect the AI agent with new orders that override its intended purpose.
It should also have a mechanism to monitor output before sending it to the customer. This mechanism should filter out offensive language, prevent the leak of sensitive data and ensure that responses are limited to the scope of what’s appropriate for the contact center.
Limiting the AI agent’s function
Deploying an AI agent with a narrow job function helps ensure both safety and good performance. Your solution provider should use that narrow definition to limit what the AI agent can do by breaking down workflows into discrete tasks, providing clear instructions for each step, and building in quality checks along the way. This prevents the AI agent from taking action outside of its intended purpose.
AI agents rely on APIs to access other systems and retrieve the data necessary to resolve a customer’s issue. Instructions at each step in the workflow must be specific and unambiguous so that the AI agent interprets the information it retrieves correctly.
It’s also critical to encode processes or steps that the AI agent must follow in a particular workflow to ensure that it does not skip steps or deviate from the intended sequence of tasks. For example, an account cancellation might require a certain amount of time to process, during which the customer could incur additional charges. Simply having this information in your knowledge base will not be enough to ensure that the AI agent informs the customer of the potential charges. Your solution must provide that specific instruction to the AI agent.
Clear process for keeping a human in the loop
Most AI solution providers acknowledge that AI is safer and more effective when it works in collaboration with humans. But not all of them have a clear set of expectations for how AI agents and their human counterparts will work together. That could leave your CX teams without the tools and information they need to provide sufficient oversight of your AI agents.
You’ll want to find a solution provider that has prioritized the human in the loop in their product from design to implementation. The solution should include an intuitive interface that enables an expert agent to monitor multiple AI-led interactions and seamlessly step in to provide approvals or personally handle the remainder of the conversation when needed. The AI agent should be able to ask the human agent for help with a clear request and sufficient context so the agent has what they need to respond.

Transparency in how the AI agent operates
Some AI solutions are black box systems that offer no information about what the AI is doing. Their vendors ask that you simply trust that it’s okay. But without insight into what data an AI agent is using and how it’s making decisions at each step in an interaction, you’re at a disadvantage with regard to safety. It’s harder to mitigate risks and ensure compliance.
A lack of transparency should be a red flag when it comes to choosing a generative AI solution. The vendor should be able to explain in clear terms how their solution functions, including how it processes data and reaches decisions. And it should empower your contact center with tools and interfaces that enable monitoring of the AI agent’s decision making and performance.
Security boundaries
To be effective in its role, an AI agent needs to interact with other software and data systems in your organization. But it shouldn’t have free rein. Strong security boundaries are necessary to control what the AI agent is allowed to do and what information it can access.
AI agents access other systems using APIs. An API is a set of rules that enables software applications to communicate with each other to exchange data or activate functionality. Security and authentication in this API layer are critical to ensure that the AI agent can access data only for the customer it’s actively interacting with (and no one else’s), and that it cannot retrieve data it is not authorized to use.
During customer interactions, personal identifiable information (PII) is sometimes part of the conversation and is often necessary to resolve the customer’s issue. To maintain data privacy, this PII should always be redacted before the data is stored. Your AI solution provider should be able to explain how they have designed and implemented appropriate security controls to protect your business and your customers.
A proven approach to phased implementation
No matter how sound your safety strategy is, a bad implementation can create unnecessary risk. Your AI solution provider should be able to guide you through a safe and reliable process for planning, deployment and ongoing management of your AI agents. And they should have the expertise to consult with you on process changes within your organization that will improve the effectiveness and safety of your AI agent deployment.
The implementation process should start with an honest assessment of what kinds of customer interactions you can automate with an AI agent, and a methodology for choosing the specific use cases it would be best to target first. And the implementation plan should include rigorous testing procedures to ensure that the system behaves as expected. The vendor should work closely with your team to define a test suite of expected behaviors and outcomes that ensures the AI agent follows your internal policies, even in nuanced cases.
You can realize value with generative AI agents – and protect your business at the same time
Balancing value with risk can be a complex calculation with any new technology, and generative AI agents are no exception. In fact, as they redefine what’s possible in the contact center, they’re changing the terms of that value/risk equation altogether. They can already automate what was previously impossible. And as innovation continues, the value they offer will only increase. Generative AI agents are poised to deliver unprecedented returns with better customer service, improved operational efficiency and substantial cost savings.
The open-ended nature of generative AI does create new risks, which need to be considered. But those risks are manageable. With a clear understanding of where the risks originate, a solid strategy for mitigating those risks within your organization, and an AI provider that builds safety mechanisms into their solutions, you can realize significant value – while protecting your business.