Jonathan Rossi
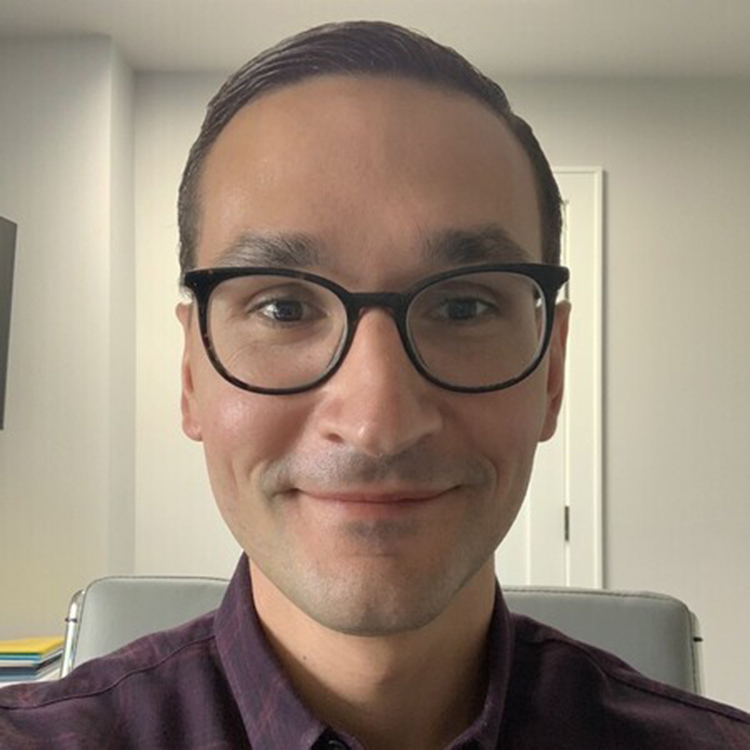
Jonathan Rossi is a Senior Customer Experience Manager at ASAPP, where he partners with our largest customers to use ASAPP data and technology to solve challenging business problems. Jonathan holds a bachelors degree in Economics from Harvard College and an MBA from Columbia Business School. When he's not running outdoors, Jonathan can be found near a couch with his dog Kit, scheming on where they'll find their next delicious meal.
How do you know if ML-based features are really working?
It doesn’t take a rocket scientist (or a machine learning engineer) to know that customer service representatives need the right tools to do their job. If they don’t have them, both rep and customer satisfaction suffer.
As a Senior Customer Experience manager at ASAPP, I’ve spent the last several years partnering with some of the world’s largest consumer companies to improve their contact center operations and overall customer experience. As they adopt our technology, they’re eager to see the investment’s impact on operational performance. Measuring success typically starts with examining how agents interact with the system.
What happens when we empower agents with better technology? Do we create a positive feedback loop of happier employees and more satisfied customers? How can we track and learn from our best performers? Can usage be correlated with other important agent efficiency and success metrics? These are the types of questions my team seeks to answer with our customers as we evaluate a program’s augmentation rate.
Our suite of integrated automation features, including AutoSuggest, AutoComplete, and AutoPilot, use machine learning to augment agent activity. The system recommends what reps should say or do during the course of an interaction with a customer. The machine learning models improve with usage—which in the contact center space can be millions of interactions per month. We work with our customers to measure the impact of these technologies on their operations and KPIs through our augmentation rate metric, which evaluates the percentage of messages sent by agents that were suggested by our algorithms.

A recent analysis found that each time one of our customers’ agents used a suggested response instead of typing freehand, they saved ~15 seconds. The time savings added up fast.
Jonathan Rossi
Augmentation rate isn’t a common metric (yet). But it offers tremendous value as an indicator of how well the technology is being adopted, and therefore, the likelihood it will have an impact on performance outcomes.
From my experience, the top three things operators should know when utilizing this metric are:
- Iteration over time:
- Augmentation rate offers guidance on:
- How well the system is augmenting agent responses and learning through data;
- How well reps are trained and coached to use the tools available to them inside our platform.
- Both the system’s model and rep training can be calibrated and optimized to continually increase the effectiveness of these features.
- Workforce Management (WFM) Implications:
- The top-level augmentation metric is helpful in measuring overall program health, but looking at usage across groups and individuals can also be extremely informative for supervisors and coaches when assessing agent and cohort performance.
- We’ve found correlations between increased augmentation usage, AHT reduction, and improved CSAT for high-performing reps.
- Incentives matter.
- If you incentivize a workforce on this metric alone, there can be adverse effects. We’ve seen reps attempt to “game the system” by always using a suggested message, then editing the response before sending. This actually increases conversation duration and decreases productivity compared to not using the features in the first place.
- Augmentation should be one of multiple metrics that go into agent performance incentives (alongside others like CSAT, throughput, and resolution rate).
By studying augmentation rates at customer companies, we’ve been able to see exactly where agents get the most benefit from integrated automation and where pain points still exist. From that knowledge, ASAPP has begun building new capabilities to increase the impact ML can have on modern workforces. For example:
- Our product team is developing additional AutoPilot features (like AutoPilot Greetings) that will automate the beginning of conversations, so reps can focus on the “meat” of an interaction and better assist the customer.
- We know that both agents and customers prefer personalized conversations. Our research and product teams are tackling this problem in two ways. First, we incorporate custom responses into our platform, enablinging reps to curate a repository of preferred messages to send to customers. This allows for agents to use suggestions in their own voice. Second, as we get more malleable in leveraging customer-specific data throughout our platform, we’re embedding more personalized customer information directly into these suggestions.
Early feedback on these additions to our augmentation features have been overwhelmingly positive from both agents and operators. Like our machine learning models, we aim to iteratively improve our product capabilities over time through usage and impact analysis, working with our customers to radically increase rep satisfaction and efficiency—which ultimately benefits the customer experience.