Introducing the Spoken Language Understanding Evaluation (SLUE) benchmark suite
Progress on speech processing has benefited from shared datasets and benchmarks. Historically, these have focused on automatic speech recognition (ASR), speaker identification, or other lower-level tasks. However, “higher-level” spoken language understanding (SLU) tasks have received less attention and resources in the speech community. There are numerous tasks at varying linguistic levels that have been benchmarked extensively for text input by the natural language processing (NLP) community – named entity recognition, parsing, sentiment analysis, entailment, summarization, and so on – but they have not been as thoroughly addressed for speech input.
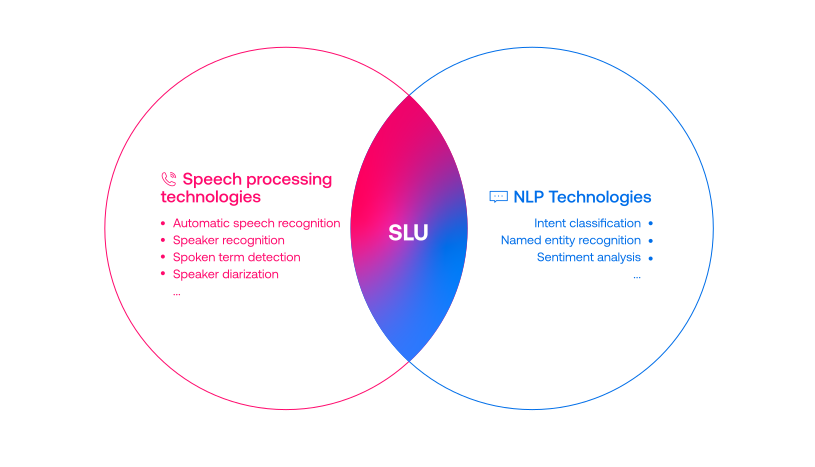
Consequently, SLU is at the intersection of speech and NLP fields but was not addressed seriously from either side. We think that the biggest reason for this disconnect is due to a lack of an appropriate benchmark dataset. This lack makes performance comparisons very difficult and raises the barriers of entry into this field. A high quality benchmark would allow both the speech and NLP community to address open research questions about SLU—such as which tasks can be addressed well by pipeline ASR+NLP approaches, and which applications benefit from having end to end or joint modeling. And, for the latter kind of tasks, how to best extract the needed speech information.

For conversational AI to advance, the broader scientific community must be able to work together and explore with easily accessible state-of-the-art baselines for fair performance comparisons.
We believe that for conversational AI to advance, the broader scientific community must be able to work together and explore with easily accessible state-of-the-art baselines for fair performance comparisons. A present lack of benchmarks of this kind is our main motivation in establishing the SLUE benchmark and its suite.
The first phase of SLUE
We are launching the first benchmark which considers ASR, NER, and SLU with a particular emphasis on low-resource SLU. For this benchmark, we contribute the following:
- New annotation of publicly available, natural speech data for training and evaluation on new tasks, specifically named entity recognition (NER) and sentiment analysis (SA), as well as new text transcriptions for training and evaluating ASR systems on the same data.
- A benchmark suite including a toolkit for reproducing state-of-the-art baseline models and evaluation, the annotated data, website, and leaderboard.
- A variety of baseline models that can be reproduced to measure the state of existing models on these new tasks.
- A small size labeled dataset to address a new algorithm and findings for low-resource SLU tasks
SLUE covers 2 SLU tasks (NER and SA) + ASR tasks. All evaluation in this benchmark starts with the speech as input whether it is a pipeline approach (ASR+NLP model) or end-to-end model that predicts results directly from speech.
The provided SLUE benchmark suite covers for downloading dataset, training state-of-the-art baselines and evaluation with high-quality annotation. In the website, we provide the online leaderboard to follow the up-to-date performance and we strongly believe that the SLUE benchmark makes SLU tasks much more easily accessible and researchers can focus on problem-solving.

Current leaderboard of the SLUE benchmark
Why it matters
Recent SLU-related benchmarks have been proposed with similar motivations to SLUE. However, those benchmarks cannot perform as comprehensively as SLUE due to the following reasons:
- Some of their tasks already achieve nearly perfect performance (SUPERB, ATIS), not enough to discriminate between different approaches.
- Other benchmark datasets consist of artificial (synthesized) rather than natural speech (SLURP), which don’t recreate real-world conditions
- There is no training audio available while only providing audio for evaluation (ASR-GLUE)
- Other benchmark datasets use short speech commands rather than longer conversational speech (SLURP, FSC)
- Have license constraints limiting their industry use (Switchboard NXT, FSC)
SLUE provides a comprehensive comparison between models without those shortcomings. An expected contribution to the SLUE benchmark would
- Track research progress on multiple SLU tasks,
- Facilitate the development of pre-trained representations by providing fine-tuning and eval sets for a variety of SLU tasks,
- Foster the open exchange of research by focusing on freely available datasets that all academic and industrial groups can easily use.
Motivated by the growing interest in SLU tasks and recent progress on pre-trained representations, we have proposed a new benchmark suite consisting of newly annotated fine-tuning and evaluation sets, and have provided annotations and baselines for new NER, sentiment, and ASR evaluations. For the initial study of the SLUE benchmark, we evaluated numerous baseline systems using current state-of-the-art speech and NLP models.
This work is open to all researchers in the multidisciplinary community. We welcome similar research efforts focused on low-resource SLU, so we can continue to expand this benchmark suite with more tests and data. To contribute or expand on our open-source dataset, please email or get in touch with us at sshon@asapp.com.
Additional Resources
- Attend our ICASSP 2022 session
- SPE-67.1: SLUE: NEW BENCHMARK TASKS FOR SPOKEN LANGUAGE UNDERSTANDING EVALUATION ON NATURAL SPEECH
- Presentation Time: Thu, 12 May, 08:00 – 08:45 New York Time (UTC -4)
- Attend our Interspeech 2022 special session “low-resource SLU”
- September 18-22, 2022, Incheon, South Korea
- Paper
- SLUE Benchmark Suite (Toolkit and dataset)
- Website and leaderboard
- Email me (sshon@asapp.com) or get in touch with us @ASAPP.