Blog
Will the real AI agent please stand up
Not all AI agents can deliver in the contact center
The adoption of autonomous AI agents is steadily increasing in contact centers, where they offer customers quicker service 24/7 and keep human agents’ queues manageable. How well each solution delivers depends on two things: what the provider prioritizes in the customer experience and how it uses generative AI to power its autonomous agents.
Providing an excellent customer experience consistently is a balancing act of technology, humanity, and efficiency. Customers want reliable responses and resolutions they can trust. At the same time, they want to avoid rigid experiences that don’t adapt to the realities of human conversation and real-world customer service. And let’s not forget speed and convenience.
Every AI solution provider balances these customer expectations in its own way. But the current crop of AI agents tend to fall into three categories, and I would argue that only one of them is truly an autonomous AI agent. The other two fall short, each in their own way.
Category #1: The better bot
These solutions prioritize consistency and safety, but lack flexibility and do not take advantage of generative AI’s ability to plan and problem-solve.
Like traditional bots, these solutions rely on deterministic flows rather than leveraging generative AI’s ability to reason its way through the interaction. In other words, they run on rails and cannot deviate from the pre-determined paths. They can use retrieval augmented generation (RAG) to gather the information they need to craft a response. But the use of large language models (LLMs) is limited in these solutions. They typically use LLMs only to understand the customer, determine intent, and choose the deterministic flow that best fits the customer’s needs.
Here’s a typical example of how this solution breaks down in a customer conversation. This is an excerpt from an actual interaction in which the caller is trying to schedule a dental appointment.
Despite the fluid conversation, the overall experience is rigid. When a customer switches topics or the interaction otherwise deviates from the planned conversation flows, the solution has a hard time adapting. That often leads to dead ends and a lack of resolution for the customer.
Overall, it feels like talking to a bot. A better bot, yes. But still a bot.
Category #2: Flexible with everything, including the facts
Solutions in this category prioritize flexibility and fluid conversation. That combination can make them feel more human. In a demo, they shine. But without sufficient grounding and safety measures, the open-ended nature of the AI leads to misinformation.
These solutions rely on the reasoning capabilities of LLMs. But instead of seeing their output as an ingredient that needs to be combined with other technologies to maintain safety and reliability, they treat the LLM’s output as the final product. That leads to a more natural feeling conversational flow. Unfortunately, dealing with an AI solution that lacks guardrails is a little like dealing with a pathological liar. Sometimes, it makes things up – and it’s hard to tell when it’s doing that.
Here’s a typical example of how this type of solution breaks down in a customer conversation. As with the previous example, a patient is trying to schedule a dental appointment.
And here’s the catch – there’s no one named Dr. Harris at this practice.
The conversation flowed well, but the solution just scheduled an appointment with a dentist who doesn’t exist. And to make matters worse, it seemed to suggest that the caller could expect to have a non-existent discount applied.
These types of solutions are inconsistent in their responses. Sometimes they’re accurate, and other times they’re misleading. And if you call again with the same questions, you just might get a different result. And you won’t necessarily know what’s true.
Category #3: A solution that lives up to the name AI agent
This last category combines the safety and accuracy of the “better bot” with the open-ended nature of the solutions that prioritize flexibility. The result is a richer, more accurate, and more satisfying customer experience.
These types of agentic solutions leverage the full capabilities of LLMs to engage in free-flowing conversations, determine customers’ needs, and take action to resolve their issues on the fly. They use multiple models to plan, reason, take action, and check output for quality and safety. In these solutions, the output of the LLMs is an ingredient, not the final product. In addition to the LLM, these solutions incorporate a robust set of safety mechanisms to keep the AI agent on track, within scope, and grounded in your designated sources of truth. These mechanisms catch potential safety and security issues in the caller’s inputs, and prevent inaccurate information from being shared in a response. When this type of AI agent does not know the correct answer, it says so. And it can transfer the caller to a human who can pick up where the AI agent left off.
An AI agent in the contact center that can successfully handle and resolve a wide range of Tier 1 conversations and issues on its own offers significant value. We’re still in the early days of these AI agents, but they can already automate complex interactions, from fluid conversations, through flexible problem-solving, to resolutions that satisfy customers. They won’t make the types of mistakes we saw in the examples above. And they’ll only get better from here.
So, what’s the catch? It can be difficult to differentiate between these categories of solutions to identify which ones live up to the name AI agent. Here’s one clue to look for – at each turn in the conversation, an AI agent worthy of the name can be a little slower to respond than the other types of solutions. It’s taking the time to ensure safety and accuracy. So, it’s a good idea to maintain some healthy skepticism when you encounter an especially cool conversational demo. You’ll want to push the solution to see whether it makes things up or has sufficient safety mechanisms to give reliable, grounded responses.
The solutions that combine natural conversation and the ability to action on the customer’s behalf with robust safety mechanisms are the future of the contact center. They deliver fluid experiences with the flexibility to adapt in the moment, while maintaining safety and accuracy. And as fast as AI solutions are improving, the response speed will come, probably sooner than we expect.
Is the human in the loop a value driver? Or just a safety net?
The latest crop of AI agents for the contact center can engage in fluid conversation, use reasoning to solve problems, and take action to resolve customers’ issues. When they work in concert with humans, their capabilities are maximized. That makes the human in the loop a critical component of any AI agent solution – one that has the potential to drive significant value.
Most solution providers focus on the human in the loop as both a safety measure and a natural escalation point. When the AI fails and cannot resolve a customer’s issue, it hands the interaction to a human agent.
Many contact center leaders see this approach as appropriately cautious. So, while they steadily expand automated self-service options, they tend to keep human agents front and center as the gold standard for customer service.
But here’s the catch: It also imposes significant limitations on the value AI agents can deliver.
Fortunately, there’s a better approach to keeping a human in the loop that drives the value of an AI agent instead of introducing limitations.
The typical human-in-the-loop roles
You probably won’t find a solution provider who doesn’t acknowledge the importance of having a human in the loop with a generative AI agent. But that doesn’t mean they all agree on exactly what that human should be doing or how the solution should enable human involvement. For some, the human in the loop is little more than a general assurance for CX leaders that their team can provide oversight. Others use the term for solutions in which AI supports human agents but doesn’t ever interact with customers.
Beyond these generalities, most solutions include the human in the loop in one or more of these roles:
- Humans are directly involved in training the AI. They review performance and correct the solution’s output during initial training so it can learn and improve.
- Humans continue to review and correct the AI after deployment to optimize the solution’s performance.
- Humans serve as an escalation point and take over customer interactions when the AI solution reaches the limits of what it can do.
The bottleneck of traditional escalation
Involving members of your team during deployment and initial training is a reliable way to improve an AI agent’s performance. And solutions with intuitive consoles for ongoing oversight enable continued optimization.
But for some vendors, training and optimizing the AI is largely where the humans’ role ends. When it comes to customer interactions, your human agents are simply escalation points for when the AI agent gets stuck. The customer experience that generates is a lot like what happens when a traditional bot fails. The customer is transferred, often into a queue where they wait for the next available agent. The human in the loop is just there to pick up the pieces when the AI fails.
This approach to hard escalations creates the same kind of bottlenecks that occur with traditional bots. It limits containment and continues to fill your agents’ queues with customers who have already been let down by automation that fails to resolve their issue.
The incremental improvements in efficiency fall short of what could be achieved with a different human-AI relationship and an AI agent that can work more independently while maintaining safety and security.
Redefining the role of the human in the loop
The first step to easing the bottlenecks created by hard escalations is to redefine the relationship between humans and AI agents. We need to stop treating the humans in the loop as a catch-all safety net and start treating them as veteran agents who provide guidance to a less experienced coworker. But for that to work, the AI agent must be capable of working independently to resolve customer issues, and it has to be able to ask a human coworker for the help it needs.
With a fully capable autonomous AI agent, you can enable your frontline CX team to work directly with the AI agent much as they would with a new hire. Inexperienced agents typically ask a supervisor or more experienced colleague for help when they get stuck. An AI agent that can do the same thing is a more valuable addition to your customer service team than a solution that’s not much more than a better bot.
This kind of AI agent is able to enlist the help of a human whenever it
- Needs to access a system it cannot access on its own
- Gets stuck trying to resolve a customer’s issue
- Requires a decision or authorization by policy
The AI agent asks the human in the loop for what it needs – guidance, a decision, information it cannot access, or human authorization that’s required by policy. Once the AI agent receives what it needs, it continues handling the customer interaction instead of handing it off. For added safety, the human can always step in to speak with the customer directly as needed. And a customer can also ask to speak to a human instead of the AI agent. In the ideal scenario, you have control to customize the terms under which the AI agent retains the interaction, versus routing the customer to the best agent or queue to meet their needs.
Here is what that could look like when a customer calls in.
The expansive value of human-AI collaboration
With this revised relationship between humans and AI agents, the human in the loop amplifies the impact of the AI agent. Instead of creating or reinforcing limitations, your human agents help ensure that you realize greater value from your AI investments with these key benefits:
1. Faster resolution times
When an AI agent can request and get help – and then continue resolving the customer’s issue – customers get faster resolutions without transfers or longer wait times. That improves First-Contact Resolutions (FCR) and gets customers what they need, faster.
2. More efficient use of human agents
In the traditional model, human agents spend a lot of time picking up the pieces when AI agents fail. With a collaborative model, agents can focus on higher-value tasks, such as handling complex or sensitive issues, resolving disputes, or upselling services. They are not bogged down by routine interactions that the AI can manage.
3. Higher customer satisfaction
Customers want quick resolutions without a lot of effort. Automated solutions that cannot resolve their issues leave customers frustrated with transfers, additional time on hold, and possibly having to repeat themselves. An AI agent that can ask a human coworker for help can successfully handle a wider range of customer interactions. And every successful resolution improves customer satisfaction.
4. Scalability without compromising quality
The traditional model of escalating to humans whenever AI fails simply doesn't scale well. By shifting to a model where AI can consult humans and continue working on its own, you ensure that human agents are only involved when they are uniquely suited to add value. This makes it easier to handle higher volumes without sacrificing quality or service.
5. Continuous learning to optimize your AI agent
Interactions between the AI agent and the human in the loop provide insights on the APIs, instructions, and intents that the AI needs to handle similar scenarios on its own in the future. These insights create the opportunities to continue fine-tuning the AI agent’s performance over time.
Generating value with the human in the loop
By adopting a more collaborative approach to the human-AI relationship, contact centers can realize greater value with AI agents. This new model allows AI to be more than just another tool. It becomes a coworker that complements your team and expands your capacity to serve customers well.
The key to implementing this approach is finding an AI solution provider that has developed an AI agent that can actively collaborate with its human coworkers. The right solution will prioritize flexibility, transparency, and ease of use, allowing for seamless integration with your existing CX technology. With this type of AI agent, the humans in the loop do more than act as a safety net. They drive value.
Why wait? JetBlue’s blueprint for leading AI-driven CX transformation
What if the biggest obstacle to improving customer service isn’t technology, but the fear of jumping in before you're fully ready? In this final installment of our three-part series on JetBlue’s approach to generative AI in its contact center, Shelly Griessel, VP of Customer Support, shares her team's forward-thinking strategy for customer support and explores the realities of deploying ASAPP’s GenerativeAgent® (JetBlue’s Amelia 2.0). Her message is clear: don’t wait for the perfect conditions to start — the time to act is now, or risk falling behind, especially from a cost perspective.
You can also watch the full discussion. [link to full Wistia video].
Read Part 1, JetBlue’s CX journey: tackling challenges in an evolving industry.
Read Part 2, How JetBlue aligns costs, culture, and AI for CX success.
* Minor edits have been made to the transcript for clarity and readability.
Embracing generative AI to boost resolution and satisfaction
Dan: The way ASAPP thinks about it is that we're trying to build is something that helps improve the performance of agents, but also candidly to reduce the number of agents or labor hours or tier one interactions, whatever term you're using.
When you and I were speaking, you put it into a similar construct. And when you're thinking about AI, you're thinking about tech. You're looking at how I can improve and accelerate the performance of my crew members (JetBlue’s contact center agents), and how to reduce the pizza pie, so to speak, of the number of agents.
So take us through that. Because you are partnered with ASAPP, you’re using us for digital, for chat essentially, and live agent interactions all through digital. And then you've just recently deployed GenerativeAgent, or Amelia.
Take us through that journey of how you're improving the performance of an agent or accelerating the performance. And then you've introduced GenerativeAgent, or Amelia 2.0 recently.
Shelly: So the plan has been all along that we have to make the pizza pie smaller because that's how you bring costs down. We have to bring volume down. You have one shot at getting it right because if you don't get it right, then the customer will call back again and again. I mean, I don't know about your industries, but when a customer is not happy in an airline situation, they will call you back six, seven, eight, nine, ten times.
And that wastes money. So, the idea has always been that first contact resolution is a big deal for us, followed by CSAT.
I will never say we don't care about handle time, but we manage handle time as a separate entity altogether. If we are able to just shrink the pie by making the crew members more effective, we can push more of the really simple stuff to Amelia, and she will deal with it. I think now that we've got generative AI going, we really want to accelerate what she's able to do, and to have more of the bigger conversations with customers.
Understanding customer intents to optimize support
Shelly: I don't believe that Amelia should have the personality of being super empathetic because everybody knows she's a bot. So you have to be very careful that it still remains authentic, and she's not gonna ever be super authentic.
I think that the customer wants to get the job done as fast as possible, and get the right resolution that they're looking for. So we have to just keep on looking at understanding why customers are contacting us, and ASAPP has done an amazing job for us to explain the intent of our customers.
Once you understand that better, you can actually start looking at your product and say we need to make changes in the product. Why do they keep on calling about baggage? They don't like the baggage policy? Or checking in? They don't like that policy?
ASAPP has helped us a lot to understand the intents of why customers are contacting us. But that's all technology that is helping us shrink the pie.
Nobody, no company, wants to pay tens of or hundreds of millions for customer support. They don't. They want to invest the money in brand-new aircraft, and so they should.
We have an obligation to get a whole lot smarter about it. So our strategy is very much constantly evaluating our tech stack. Is it what it's what it's still needed? Do we provide them with enough information to be able to do the job? Like guided call flows. And making sure that crew members understand this is how it's going to help you versus anything else.
From proof of concept to progress: Teaching GenerativeAgent
Dan: I was thinking about this as you were speaking. I saw some great research. Shout out to Brian and Brooke from CMP on the research. I saw in a session yesterday around chatbots and voice bots just some dissatisfaction with customers and etcetera.
Everybody's familiar with that. When you dipped your toe into GenerativeAgent, or Amelia 2.0, what were concerns that you had going in? Because chatbots and voice bots promised a lot of the same things that you're hearing from a generative AI agent. And so what we hear a lot of is skepticism because we promised a lot, and it didn't necessarily happen.
So when you approached generative AI, how did you approach that to go, I'm going to see if GenerativeAgent, or Amelia 2.0, can actually work? And then tell us about the journey, trepidation, results, anything that you would wanna share about that.
Shelly: So we started in May when we said, okay, let's do a POC (proof of concept), and let's see how it goes.
And we had a team watching it and course correcting. I think you're familiar with the term hallucination. So she comes up with things that you go, why did you say that, Amelia? That's not true.
And then it's a matter of, okay, let's pull her back. Let's teach her how to do this differently. And I think that we've got enough – so this started in May. At that time, our containment with her was at about 9%. And then by August, she went up to as high as 21%.
And that's amazing in a very, very short period of time, and it's just a proof of concept. So it's very little volume that we're giving her, but I think that we now need to double down on this. I want to fast-track teaching her. I think that this has to come from taking some of our best crew members in the company and watching her and saying, “No, take that option away.” So there are certain things, for instance, that we learned that we don't want her to do.
There’s so much pressure on airlines at the moment to get your refund policies right. So the DOT is all over us. We can't let her make decisions on refunds. So we say, okay. Put that out of scope. What else is a hot topic? Like ADA, hot topic. Wheelchairs, hot topic. You have to keep that stuff out.
And I think that it's just going to take a little bit of time blending humans with teaching her on the areas that she can absolutely start knocking out of the park, and we'll get there. I think it just has to be this relationship made between humans and Amelia to learn.
I think that some of the companies that are getting some good success with it are taking a bot, whatever bot they have, and let the bot learn from a human. So I think that matching what great crewmembers can do with the bot is for us looking like that's going to be the future.
Start now even if you are not ready – or risk being left behind
Dan: A lot of the questions that we hear at ASAPP are, “I'm not ready for a GenerativeAgent experience because I've got knowledge base issues or technical debt” or any of those things.
If you were to give any advice to this audience about a place to start this journey – for people who are wanting to start on this AI journey but aren't ready to, like, deploy some sort of GenerativeAgent, where could they start? How do you evaluate?
Shelly: Your environment is never going to be right and ready. It's never. I mean, come on. For all of us that have been in customer support areas forever, every year we plan all the things that I'm going to do. And before you know, it's the end of the year. And I didn't do 50% of it because why? Because we come in and there's a new drama.
I think that the time is never right. I think that for this, in my mind, you have to jump in because I think if you don't, you're going to be left so far behind, especially from a cost perspective.
I don't think it's just airlines that are under the pressure for forecasts at the moment. We're going through budgets right now, and it's tough. Everybody wants customer support to cost nothing, and please make sure the customer is really happy still.
Don't spend any money, but don't you dare let that NPS go down.
I think we all know that, and that's why I say you've got to jump in and say, what have you got to lose? If you put the boundaries around it, what have you got to lose? You really don't.
So I don't think that you have the luxury of waiting for everything to be ready and perfect. You have to go, okay, I'm ready now. Now I can do it.
I think you're gonna be left behind if you do.
Read Part 1, JetBlue’s CX journey: tackling challenges in an evolving industry.
Read Part 2, How JetBlue aligns costs, culture, and AI for CX success.
How JetBlue aligns costs, culture, and AI for CX success
In part two of our blog series on JetBlue’s approach to customer service, we dive into the financial and labor dynamics that shape the airline’s contact center operations. Shelly Griessel, VP of Customer Support at JetBlue, offers a candid look at the factors driving up costs, from first-contact resolution challenges to the role of AI in improving efficiency. But it’s not just about numbers—JetBlue is deeply committed to supporting their workforce, investing in training, and fostering a culture where employees feel valued and empowered. In this post, we’ll explore how GenerativeAgent®, what JetBlue calls Amelia 2.0, is not just a tool for improving customer interactions, but a vital partner in alleviating burnout and keeping agents engaged.
Read Part 1, JetBlue’s CX journey: tackling challenges in an evolving industry.
Read Part 3, Why wait? JetBlue’s blueprint for leading AI-driven CX transformation.
* Minor edits have been made to the transcript for clarity and readability.
Understanding Cost Drivers in Customer Service
Dan: On cost reduction in the contact center, some will say, “okay, we're trying to reduce or maintain costs.” When you think of the financial aspect that either you or your peers are seeing, what is driving up that cost? Any insight that you have on what's affecting that?
Shelly: What's driving up the cost is that if you don't keep FCR at the highest possible level, customers will keep on calling back. And more volume means the cost of calls goes up.
So you have to try and find a way of doing two things in the customer contact center environment going forward. For us, it is bringing down the cost per call, and a big part of that is containment through conventional chat or now with more progressive, what we call Amelia 2.0 (powered by ASAPP’s GenerativeAgent).
We call our bot Amelia (after Amelia Earhart) because we had to give her a name.
We really like her. She shows up every day. She's got no absenteeism problems, never wants PTO, and she just shows up every day, and she's always friendly. She's, like, always friendly.
The Role of AI in Enhancing Customer Interactions
Shelly: So now we've got Amelia 2.0, which is gen AI, and she's a little bit more conversational, sometimes too much, but we're getting her there.
And I think that that is the next evolution.
We have to free crew members (JetBlue’s contact center agents) from having to deal with very basic stuff, and frankly, they get bored with it.
Our tenure is extremely long at JetBlue. The average tenure is about ten and a half years; they don't leave. But also the majority of our crew members are part-time.
So they work anything between fifteen and thirty hours a week. So that also helps with the lack of burnout. They don't burn out, and that's why.
But gen AI has got a massive role to play in this. It has a massive role to play in it.
Dan: When you're approaching the big topic of AI – something that’s so ubiquitous now that it's become very generic and losing a lot of its meaning and power – how are you and the team approaching those waters in the contact center space? What are the concerns and the outcomes that you're trying to get?
Shelly: So when we started really ramping up AI, there was obviously a massive fear by our crew members about “it's gonna take my job away.” And that was a very real fear for them.
And then they started realizing that she (Amelia 2.0) actually covers the shifts that they don't want to do. So Amelia became very handy. She would work weekends, and she would work through the night. So from that perspective, it became less of a threat for them because they knew that she was complementing them.
And our containment is extremely high. We started two years ago in the low 30s – 35, 36% containment. And now we're sitting at between 68% and 70% containment.
It just never gets past Amelia. Amelia keeps it. But when a call actually eventually comes over to the crew member, all the hard work has kind of been done already, and they can step in and just start making decisions that the customer is looking to be made. So for us, we've embraced AI as a company. Crew members are still more afraid of “will a BPO take over my job?” They're all more afraid of that than they are of AI now.
Addressing Labor Concerns in the Age of AI
Dan: When we talk about how agents are gonna lose jobs because of AI, I'm wondering why we aren't talking more about how agents are removing themselves from the job themselves and in record numbers. I think we are at 52-62% average turnover, major absenteeism, and the majority of contact center leaders are saying they are having a hard time recruiting.
I'm really interested in your thoughts on AI when it comes to this big issue of labor in the contact center and the high cost and absenteeism, and how contact centers are just dealing with that.
Shelly: We are proud of the fact that we don't lose people. But I think it's got a lot to do with the part-time model that we run. In fairness, there's a massive burnout level.
If you take call after call after call - and customers, let's be honest, they don't call in to say, ”way to go,” “I had a great flight,” “my flight was on time.” That's a given.
They don't. I mean, so every single call, call after call after call.
So we obviously manage it through the fact that they don't have these 40-hour week shifts, and they work part-time, so it makes for life to be a whole lot easier. But we also have a responsibility – and we spend a lot of time on culture. So we double down on culture. We watch our ratios of crew members to supervisors.
Maintaining Relevance in a Changing Workforce
Shelly: I was sharing with you earlier how much time we spend with crew members – what we call the PDRs, which is protecting the direct relationship that we have with them. It's a big deal for us. And we explain to them the why behind BPOs, the why behind Amelia. And the more they understand it, the better.
But the other obligation that we have in this industry is to make crew members or agents, wherever they are, relevant in five years from now because they won't be relevant if we don't make the effort. It's kind of our responsibility to do it.
And we have to teach them different scenarios of how to deal with de-escalation or really complicated problems. We have to, and that is where technology comes into it. Technology becomes their friend.
So I think we have a massive obligation and a responsibility to keep them relevant in the new world, and the communication has to be so big and so wide open to bring them along.
The worst thing that we can do is to make them feel disenchanted and they stay. Because if they stay, your customers will feel the impact of their unhappiness.
You've got an obligation. Employee engagement scores are huge in JetBlue. It's very, very big.
So we spend a lot of time on that, but I think that embracing technology as a part of it, we've never shied away from the fact that Amelia is there and this is what she does. These were her stats. We give them her stats on a daily basis because she's part of the team.
It's weird. She's not really a person, you know that.
Dan: Amelia is ASAPP's GenerativeAgent, but when she talks about it as Amelia, I'm like, yeah, she's part of the team. This nice woman in the front row said, “I wanna be Amelia's friend now.”
Fostering a Valued Workforce Culture
Dan: So, to put a finer point on it, because we are gonna talk about the tech part of this and the partnership with ASAPP later. Can you touch on what you are doing in the culture that you think, “this is going to have a really appreciable effect on keeping the crew members in the job?”
Shelly: People really want to feel they’re valued in the world. The feedback we constantly get from our crew members is that this is the first place I have worked at that I don't feel like a number. I'm not a number.
They've got access, and when we say they've got access to me as the VP, that is not just empty talk. It's real.
They really do have access. Every leader in my team has to dedicate two hours a week in their calendar for any crew member to talk to them about anything. Anything. And they blocked up those views.
We have monthly what we call "directly to you” meetings, in which it's open kimono. We tell them all the good and all the bad. The company is not doing well. The company is doing well. This is where we're going. Thhis is the good and the bad.
We've got a CEO that absolutely believes in 100% transparency. There's absolutely no point in sugarcoating anything. You have to be very honest with people.
And that's how we bring 24,000 people along with us. That is the total number of crew members that we've got in the company, but that's how we bring them along and how we protect the culture. So for the customer contact center area, we are not unionized, and there's no talk of being unionized because people know that things get done faster when they come to Shelley directly or their director or their manager to say, “I'm really not happy about this.” “What are we gonna do about it?” “Oh, well, this is why I can't do anything about it.”
So we absolutely believe in a very transparent relationship with them. We tell them the good and the bad all the time.
Read Part 1, JetBlue’s CX journey: tackling challenges in an evolving industry.
Read Part 3, Why wait? JetBlue’s blueprint for leading AI-driven CX transformation.
JetBlue’s CX journey: tackling challenges in an evolving industry
Customer support is not just about answering customer questions—it's also about resolving customer issues quickly, and adapting to rapidly changing expectations. JetBlue has been on the forefront of this shift, with a model that’s built on 25 years of remote work and a deep commitment to empowering their agents. In this post, part one of a three-part blog series based on a discussion between Dan Rood, SVP Marketing at ASAPP, and Shelley Griessel, VP of Customer Support at JetBlue, we explore how the airline’s approach has adapted to the challenges of a rapidly evolving industry, where the unpredictable nature of customer needs and agents’ lives can make every day a unique experience.
Read Part 2, How JetBlue aligns costs, culture, and AI for CX success.
Read Part 3, Why wait? JetBlue’s blueprint for leading AI-driven CX transformation.
* Minor edits have been made to the transcript for clarity and readability.
The unpredictable joys of contact center work
Dan: I'd love to hear from you - what is a moment or moments that occur to you in this industry in your role that you go, “I love this job. I love this.”
Shelly: I think without a doubt, it is the unpredictability.
We all think we've got our week planned out, we've got our day planned out, but it really doesn't work like that. I think that I love, love, love the fact that I've got absolutely no idea what's going to come at me today… And the unpredictability of what's happening in your crew members' lives (that's what we call our agents).
And it makes for just constant entertaining. Sometimes it's heart-wrenching stuff that you have to deal with. So we go permanently from a state of being absolutely ecstatic about life and, like, “we're winning every day” – and then they are just heartbreaking times.
And I think that's what I love most about the job.
I don't think… this is not what I planned to do in life, but just being in contact centers for so long, I think I've been blessed to have experienced so much over the thirty-five years that I've been in the business.
Why contact center challenges persist
Dan: If we were to go back five years or ten years, the conversation in any surveys that we have in a room like this, is that three or four things happen in a contact center – controlling, or even reducing costs, and improving CSAT, are the themes over and over again. And yet, in 2024, there's a lot of evidence to say it's not necessarily getting better. If you were to just step back, why does it feel like it is not getting better?
Shelly: I think to start off with, the last four to five years have made it exponentially harder. There's something that has just clicked in the mind of consumers that they're not tolerating as much.
It feels like it is a lot harder to please. And I think that people are also more than ever before stretched for time. There's just so little tolerance for long hold times, and then they get through to somebody who is not going to wrap it up quick enough.
I think the most precious commodity in today's world is absolutely time.
I am of the belief that they want to get an answer quickly. They want to get a consistent answer. If they don't like it, then they'll phone back three or four or five or six or seven times, until they get the answer that they're looking for. That drives up costs, that drives down FCR.
It's just a lot more complicated, and I think COVID has a lot to do with it. It has also got a lot to do with how much more challenging crew members' lives are. It is harder, and the value of the job hasn't gone up. So people are not necessarily getting paid more, and they're holding down two or three jobs, which makes their lives complicated.
Remote work and customer expectations
Shelly: In the JetBlue model, our contact center crew members work one hundred percent from home, and have since the beginning for 25 years.
In addition to that, as they work from home, they were challenged with, during COVID, being alone and having no contact with anybody else. So it's been a lot worse for them.
But I do think that for customers, it is a lot more about getting through to somebody that can give me an answer really quickly, and let me be on my way. And I want to do it when I want to do it, any time of the day or night. It's just a different experience that we have to offer customers today versus what it was.
Empowering contact center roles for speed and resolution
Dan: At ASAPP, we call it abbreviating customer pain. From a performance standpoint, the main threshold that you're trying to get to is speed and obviously real resolution. Is that the strategy when it comes to keeping brand differentiation with JetBlue versus your competitors?
Shelly: Absolutely. So we do want to speed it up, and we want to make sure that we use the human intellect in a different way than what we probably did three or five years ago. Which means that we are personally investing a lot more in the training and development of people to deal with the really tough issues. It doesn't help to say to a person, “You're empowered, and you can do what you need to do.” You actually have to teach them what empowerment looks like. So there's a lot more time and resources that we're spending on evolving the role of a customer support person.
And I do believe that the footprint of humans in a contact center is going to get smaller, but I think it's gonna finally become a higher paid role, because they're going to deal with a lot more complicated matters – a lot more complicated – and we should pay them more.
So I think the footprint will get smaller, but we should be able to pay them more.
Read Part 2, How JetBlue aligns costs, culture, and AI for CX success.
Read Part 3, Why wait? JetBlue’s blueprint for leading AI-driven CX transformation.
The road ahead: What the next year will bring for AI in CX
We all hear the same thing about the future of the CX landscape every year, that it’s poised for a dramatic transformation. Most years, the steady evolution of CX tech delivers real gains for customers and businesses but falls short of true transformation. But this year seems different. As CX leaders embrace generative AI, the role of contact centers—and the agents who work within them—will undergo a profound shift. The key to navigating this colossal change will be effectively using the full range of genAI’s capabilities to improve both customer and employee experiences.
The growing complexity of AI use cases for CX
Over the next year, two things will happen in tandem – new CX use cases for AI-native solutions will continue to emerge and enterprises will shift focus to improving data curation. A whopping 95% of customer service leaders say that improving analytics, intelligence, and quality capabilities will be a bigger priority in 2025. That focus will lead to better, cleaner data. And with better data, new AI solutions will be able to tackle more complex customer interactions.
AI is already automating highly repeatable human-assisted transactions. The capabilities that power this automation, such as summarization, will be commoditized and widely deployed. At the same time, more advanced capabilities will be developed. As AI automates more complex and time-consuming tasks, the role of humans in the contact center will evolve to focus on critical interactions that build customer relationships and strengthen the brand.
Productivity improvements are already obvious early wins with AI, and that will continue. But businesses will lean into generative AI’s capacity to personalize experiences at scale. The vast majority of CX leaders (90%) say that determining the best use cases for AI will be a key focus in 2025. The blend of efficiency and personalization that new use cases offer will finally begin to transform the way companies execute across the entire customer journey.
From legacy tech to LLMs – and beyond
Legacy technologies simply aren’t up to the task of delivering the kind of service consumers expect these days. Chatbots, websites, mobile apps, and IVRs are all okay for very specific tasks, but their inability to support the dynamic, complex, and sometimes emotive needs of the customer are where LLM-native AI is a massive leap forward – when it’s deployed thoughtfully.
Here’s the catch. An open-source LLM alone cannot manage the complexity of your customer interactions. That’s a lesson some businesses have learned the hard way with some very public failures. But a system of models grounded on your business lexicon and integrated with your company’s backend systems is incredibly powerful at achieving first contact resolution. And this is where leading companies are headed, fast.
If you haven’t already moved in this direction, you would be well served to get started now by finding an AI solution partner who can implement a range of use cases tailored to your business. You can start simple and deploy use cases of increasing complexity over the next couple of years. This iterative process will ensure ongoing learning and deliver transformational customer, employee, and shareholder value.
The evolving role of human agents
In my experience, no one knows the best and worst of your company better than the agents in the contact center. They talk to the customers that use your products and services all day, every day. So, they know what’s working and what’s not. That makes them a valuable resource, one that too often remains untapped.
As the role of AI expands to handle more routine work, the role of human agents will evolve in ways that create opportunities to leverage their unique knowledge and perspective. Nearly 89% of customer service leaders say that the typical agent of the future will handle complex customer service and support interactions. Other likely aspects of the evolving agent role include participating in client retention and winback efforts (68%), contributing to knowledge management (66%), and collecting and sharing customer feedback (66%).
The expertise of your best agents makes them uniquely equipped to train and supervise AI in a way that will completely change how your contact center operates. Not only will AI resolve the systemic issues that make contact center work incredibly difficult and expensive, but it will also empower companies to create historically high efficiency and personalization. That shift will do a lot to address consumer dissatisfaction, which creates massive headwinds to growth and profitability.
To achieve this transformation, CX employees must be part of the solution from day one. It will be crucial for businesses to involve them directly in AI adoption and expansion. While some jobs, over time, will be reduced or even eliminated, new roles will also be created. Educating employees on the importance of their role with AI, upskilling those tasked with making the technology successful, and evolving incentive programs will accelerate benefits to the customer and company.
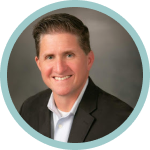
The transformational opportunity for CX leaders has never been this great. But it depends heavily on balancing the technology with the people in the right way.
- Chris Arnold, VP of Contact Center Strategy, ASAPP
Human involvement will be crucial for improving the performance of AI solutions and for ensuring safety and data security. Businesses that successfully evolve a subset of agent roles to provide supervision, human reasoning and judgment, and advanced triage will be better positioned to deliver safe and highly efficient first contact resolution at scale using AI.
Not all tech providers are equally mindful of the need for this balance of humans and AI. You’ll need to choose a partner that acknowledges the importance of people and process, rather than simply providing software. How technology is deployed matters as much as the technology itself, maybe more in this case.
Navigating this new path forward
There is a tremendous amount of noise in the market right now as companies in every industry develop their CX strategy for generative AI. This has created a massive amount of marketing from technology providers that serve contact centers, many of them painting bolt-on solutions as truly AI-native. That can make it difficult to evaluate and compare solutions and vendors. For long-term value, it will be important to identify the vendors who have deep expertise in AI and CX and have built and deployed AI-native solutions successfully. So, you’ll need to ask tough questions of vendors prior to signing a contract.
As you add generative and agentic AI solutions to your mix, don’t be afraid to start small with a plan to learn and iterate quickly. In the contact center, small changes can lead to massive benefits. Take the time to be proactive and thoughtful in choosing the right use cases to target first. Consider where the AI is strong out of the box, what data sources are available, and where pain points can be quickly eliminated. This requires more thought than simply looking at the top five call drivers. You’ll get there more quickly if you leverage the inherent strengths of the AI and invest the time and energy into creating space for the solution to learn and do what it’s built to do – meet the needs of your customers in the most effective and efficient manner possible.
The shift from cost center to value center
As we look toward the future, it's clear that the contact center is undergoing a fundamental shift. The days of seeing contact centers as mere cost centers are numbered. In the new AI-powered world, contact centers will become value centers that contribute directly to customer loyalty, retention, and revenue generation.
Traditional metrics like Average Handle Time (AHT) and First Contact Resolution (FCR) will be replaced by more holistic measures that align with the new workflows and possibilities created by AI. These new metrics will shift the focus to omnichannel resolution, customer lifetime value, and concurrency.
As more human agents shift into new roles supervising the work of autonomous AI agents, concurrency improvements will be dramatic, even with voice. The gains that will bring in productivity and efficiency will dwarf the incremental improvements we’ve seen with other recent technologies. We’re on the cusp on a radical change that will require a new approach to measuring performance. The new metrics will help drive contact centers to deliver exceptional customer experiences at scale, improving both the top and bottom lines of the business.
Thriving in this rapidly evolving landscape
The next year will see significant advancements in the contact center industry. AI will continue to reshape the way we deliver service, while human agents will play an even more critical role in driving customer satisfaction and brand loyalty. By embracing AI, empowering agents, and focusing on personalization and efficiency, businesses can position themselves to thrive in this rapidly evolving landscape. The future of customer service is here, and it’s one where technology and humans work together to deliver outstanding experiences.
Have we missed the point of empathy in CX?
Empathy in customer service doesn’t always look the way we expect. Sometimes it wears a disguise.
A few years ago, I bought my daughter a new mobile phone for Christmas. She planned to make a 3-hour drive, mostly through rural areas, to visit her cousins the next morning. We needed to get the phone working before she pulled out of the driveway.
But nothing we tried did the trick. So, late in the afternoon on Christmas day, we needed customer support.
Our service provider’s website offered two options, phone or chat. I hate chat for support, but the wait time for a phone call was more than I could commit to while getting ready for a family dinner. So, I fired up the chat and asked for help. At first, I wasn’t sure whether it was a bot or a human. I didn’t care either way as long as we got the phone working. Over nearly two hours, I alternated between the chat and my family. And in the end, the problem was fixed.
What does empathy actually look like in customer experience?
I don’t recall the agent (human after all) saying anything particularly compassionate. And yet, this was one of the most empathetic customer service experiences I’ve ever had. Here’s why:
- The interaction resolved my problem on my schedule without requiring a call or visit to the store.
- I had a clear choice between phone and chat and knew the current wait times.
- I got the problem resolved without missing Christmas dinner with my family.
The bottom line is that my service provider gave me options for how to engage and a convenient way to get what I needed.
This is how empathy sometimes wears a disguise. It masquerades as efficiency, convenience, and ease.
In an industry hyper-focused on the emotional side of empathy, we too often overlook this crucial practical side. But we shouldn’t. It matters to customers, a lot.
- 93% of customers expect their issue to be resolved on the first call
- 62% of customers would prefer to “hand out parking tickets” than wait in an automated phone tree for service or have to repeat themselves multiple times to different team members
- 80% of American consumers say efficiency is the most important factor in the customer experience, and more than half say it’s worth paying more for
The often-overlooked practical side of empathy in CX
In recent years, the CX industry has focused intently on empathy. Businesses spend time and resources to upskill agents on active listening, emotional intelligence, and expressing care and compassion. They even provide lists of empathetic phrases their agents can use. And a growing number of contact centers use AI to detect customer sentiment throughout each interaction. All of that is great. It reminds the agents that customers are human, too, and they need to hear that someone cares about their problem.
Validating a customer’s feelings is an important component of putting empathy into practice. But it’s only one component.
Caring alone doesn’t resolve a customer’s issue, and it doesn’t automatically make the process of reaching a resolution easy or convenient.
Long wait times, multiple interactions, and chatbot failures are not empathetic. Many CX leaders view those points of friction through the lens of contact center efficiency with metrics like transfer rates and digital containment. But friction also increases customer effort, which is an important component of empathy in CX. And too many contact centers deliver experiences that require a lot of customer effort – ineffective self-service, complicated IVR menus, disconnected channels, and more. An agent who says they understand your frustration can’t erase all that effort and wasted time.
Empathy in CX strategy: Are we making it too complicated?
The concept of empathy is somewhat vague and squishy, so it’s not surprising that CX leaders sometimes convert it into something else when crafting CX strategy. The problem is, they often convert empathy into the equally vague concept of customer-centricity. What does that mean? Keeping the customer front and center at all times, sure – but how? It isn’t always clear how centering the customer translates into actions and processes for the contact center to follow.
The vague nature of both empathy and customer-centricity tends to give rise to complex frameworks that attempt to make the strategy more concrete. For example, a framework might categorize elements in the CX ecosystem into systems of listening, understanding, action, and learning. Those frameworks can help shape perspectives within your business, but they still require additional translation to make them actionable for your frontline CX team.
Here’s a simpler approach. Embedding empathy into your CX strategy means consistently aiming to do these four things:
- Resolve the customer’s issue in the first interaction.
- Take up as little of the customer’s time as possible.
- Make the entire process easy and convenient.
- Treat your customers and employees like the human beings they are.
Getting to the point of empathy with generative AI
In contact centers, early AI implementations increased efficiency, but employees felt the impact more than customers. In some cases, AI deployments actually increased frustration by raising customers’ hopes with big promises of faster, more convenient service that didn’t ever materialize. Consider chatbots. Even with improved language processing, bots can’t take action to resolve a customer’s issue. So, they require time and effort from the customer but often, can’t truly help. When it comes to the practical side of empathy, they fail to deliver.
But that was then, and this is now. The technology has matured, and current implementations of generative AI are improving contact centers’ performance on both the emotional and practical sides of empathy. AI solutions increasingly take over repetitive and time-consuming tasks, freeing agents to focus more effectively on the customers they’re serving. This shift makes space to engage with more empathy across the board.
Customer-facing AI agents will generate a larger, even seismic, shift in how empathy is embedded into customer experiences. Generative AI agents can listen, understand, problem-solve, and take action to resolve customers’ issues. That ticks all the empathy boxes for me. This massive leap forward lays the groundwork for CX leaders to shift the emphasis of their AI investments toward solutions that do more than talk in a natural way.
Practical empathy that’s just a chat or call away
That Christmas a few years ago when I needed customer service, I didn’t care whether I chatted with a human or AI. I just wanted my problem resolved before my daughter left town, preferably without having to call or visit the store the next day. I got lucky that time. My service provider had agents available. But we all know that’s not always the case. With a generative AI agent ready to respond 24/7/365, the customer’s luck never runs out. Effective, efficient, and convenient service will always be just a call or chat away. For me, that’s the part of empathy in CX that too many businesses are missing today. But I suspect that’s about to change.
5 questions to ask before building your own AI solution for CX
The build vs. buy debate in software is nothing new. With the rise of large language models (LLMs), spinning up prototypes that can complete tasks has become easier than ever. However, developing an AI application for CX that’s scalable and production-worthy demands extra consideration. In this post, we’ll explore key questions to ask when deciding whether to build or buy generative AI solutions for CX.
While the right answer depends on industry, use case, and business goals, it’s important to carefully weigh your options.
Why building an AI application for CX requires extra consideration
The reason for this has a lot to do with the nature of generative AI.
Unlike most software development where the goal is to create a deterministic system with the same, expected outcome every single time, generative AI generates new information and content based on patterns, often producing varied responses that can be difficult to predict.
This variability is what makes gen AI applications so powerful, but also adds complexities in maintaining consistency and control.
Differing from standard applications where the workflow is predefined, generative AI models require continuous monitoring, training, and refinement. There is a misconception that generative AI just needs instructions. But in fact, they need much more, especially if you are training on your own data.
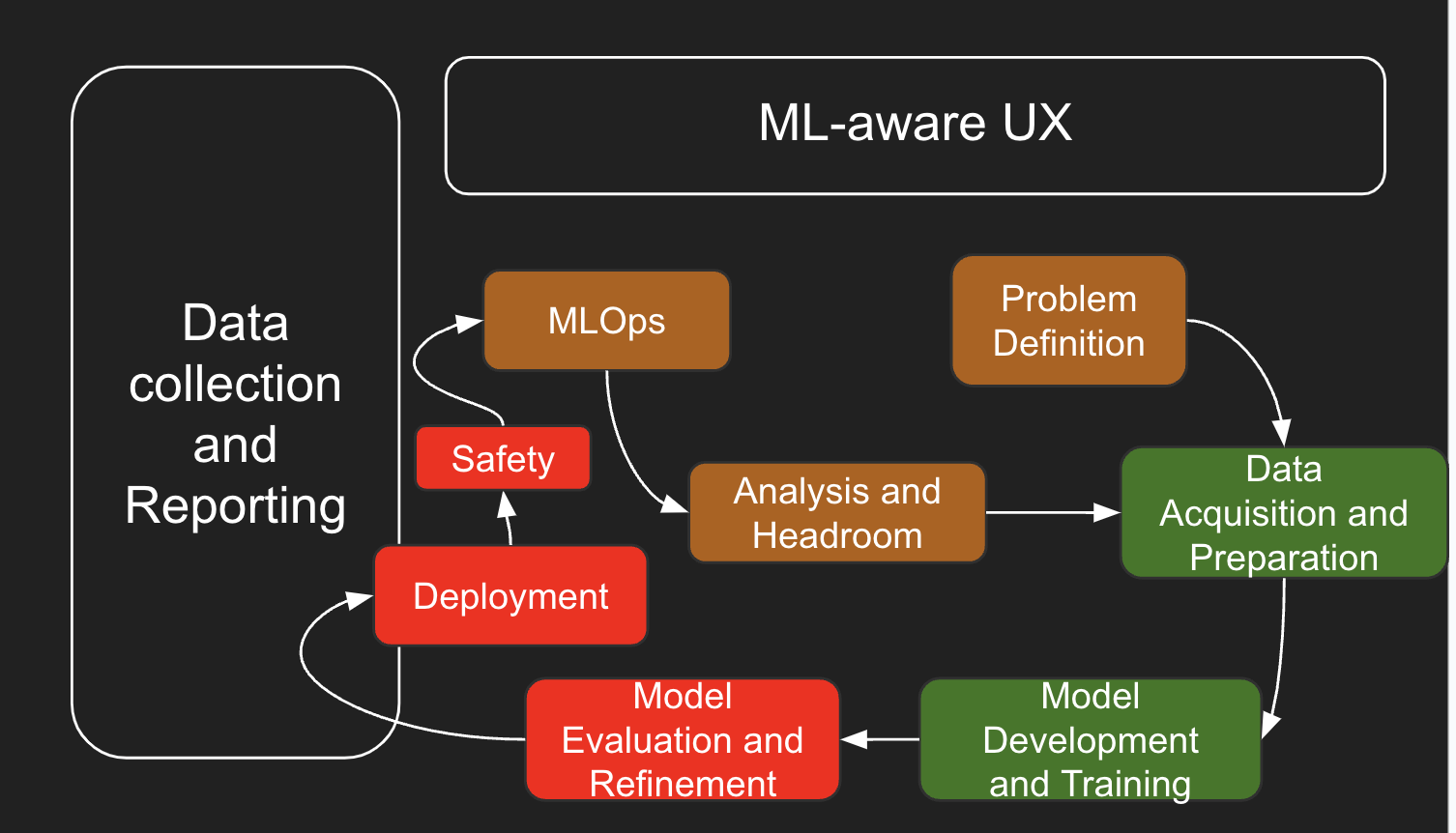
The flexibility makes them ideal for tasks that benefit from creative problem-solving or personalized engagement, but it also means that development doesn’t stop at launch. This, compounded by the fact that CX applications can ultimately impact a brand’s relationship with its customers, brings additional layers of considerations when deciding whether to build the application yourself or to partner with a vendor.
While the following is not an exhaustive list, here are some top questions you should consider when making a decision.
Is building AI applications part of your business objectives?
If building AI applications aligns with your business objectives, you’re likely prepared for both short-term and long-term investment in development and maintenance that will be absolutely critical for AI applications, ensuring the solution doesn’t become a burden on your resources.
In contrast, if developing your own AI applications isn’t a core objective, partnering with a vendor may be the more sensible approach. Vendors are equipped to provide solutions that fit your needs without the complexities of internal development, allowing you to focus on your primary business goals.
Based on McKinsey & Company’s recent estimates, building and maintaining your own foundational model could cost up to 200M with an annual recurring cost of 1-5M. Using off-the-shelf solutions or fine-tuning existing models with sector-specific knowledge can dramatically reduce this cost.
What’s your timeline for deployment?
If your deployment timeline is urgent—perhaps due to a competitor already leveraging AI solutions—working with an AI solution provider can help you hit the ground running and accelerate implementation. A vendor with solid experience in enterprise AI development will help you avoid unnecessary trial and error, minimizing risks associated with AI safety - such as AI hallucinations.
Instead, leaders should strongly consider partnering with gen AI solution providers and enterprise software vendors for solutions that aren’t very complex or [industry] specific. This is particularly critical in instances where any delays in implementation will put them at a disadvantage against competitors already leveraging these services.
- McKinsey & Company, in "How generative AI could revitalize profitability for telcos"
Do you have the internal expertise, resources, and infrastructure to build, scale, monitor, and maintain an AI solution?
While widely available large language models (LLMs) have significantly accelerated the process of building a working prototype on a laptop, this does not equate to having a production-ready, scalable solution that can effectively address your business needs [link to last mile]. Additionally, the demand for ongoing maintenance means that development does not stop after the application is launched.
Developing AI applications requires a skilled team knowledgeable in machine learning, data management, and software engineering, along with the necessary technological resources and datasets. Even choosing the right LLM to use for the best fitted use cases would require a good understanding of how various LLMs differ. Experience working with AI solutions is also crucial for successful deployment in an enterprise context.
In the last two months, people have started to understand that LLMs, open source or not, could have different characteristics, that you can even have smaller ones that work better for specific scenarios
- Docugami CEO Jean Paoli, in CIO “Should you build or buy generative AI”
If your organization lacks this expertise or the infrastructure to support such a project - not just at the prototype stage, but also to scale, monitor, and maintain the solution in the future - it may be more prudent to consider vendor solutions that can provide the capabilities you need without overextending your internal teams.
Also consider, if problems arise, do you have the manpower and expertise to investigate the root cause and implement long-term fixes, or will you be crossing your fingers and hoping the issues don’t recur? A strong internal team capable of addressing challenges as they emerge is necessary for ensuring the reliability and effectiveness of your AI application for CX. Without this capability, you risk operational disruptions and even diminishing trust in your brand.
What ROI do you expect from your AI application? And how comfortable are you with the associated risks?

When considering your expected return from an AI investment, it’s essential to balance potential returns with the associated risks.
Agent-facing projects generally carry lower risks, as the AI solution won’t directly interact with customers. This allows for more trial and error, with agents able to provide feedback on the AI application’s performance. That said, such solutions might yield only incremental gains in agent productivity with less or no impact on the customer experience, and do not take advantage of the full capabilities of gen AI.
In contrast, customer-facing gen AI applications can offer a much better return because they can directly improve customers’ ability to self-service and, in some cases, resolve their issues directly. Here are the kinds of results a customer got when deploying generative AI agents with the capability to resolve Tier-one tasks.
Allowing AI to handle a broader range of tasks introduces added complexity. While there are risks—such as AI hallucination, where the system may generate incorrect or irrelevant responses—these challenges can be managed with the right approach. Having a strong internal team that can tackle this, or choosing an experienced vendor with a well-informed strategy for handling AI behavior, will ensure guardrails are placed around customer-facing interactions — so you can get the most out of your AI applications with confidence.
Making a thoughtful decision
Ultimately, the choice between building or buying an AI solution should align with your organization’s long-term vision. Each option carries its own set of challenges and opportunities, and taking the time to assess your specific needs can set the stage for success.
Considering the evolving landscape of AI, it's not just about deploying technology, but also ensuring that it fits well into your operational framework and readies you for the future. With careful evaluation, you can make a choice that enhances your customer experience. Whether you decide to build or partner, the key is to stay focused on your goals and embrace a strategic approach to generative AI applications.
Preventing hallucinations in generative AI agent: Strategies to ensure responses are safely grounded
The term “hallucination” has become both a buzzword and a significant concern. Unlike traditional IT systems, Generative AI can produce a wide range of outputs based on its inputs, often leading to unexpected and sometimes incorrect responses. This unpredictability is what makes Generative AI both powerful and risky. In this blog post, we will explore what hallucinations are, why they occur, and how to ensure that AI responses are safely grounded to prevent these errors.
What is a Hallucination?
Definition and Types
In the context of Generative AI, a hallucination refers to an output that is not grounded in the input data or the knowledge base the AI is supposed to rely on. Hallucinations can be broadly categorized into two types:
- Harmless Hallucinations: These are errors that do not significantly impact the user experience or the integrity of the information provided. For example, an AI might generate a slightly incorrect but inconsequential detail in a story.
- Harmful Hallucinations: These are errors that can mislead users, compromise brand safety, or result in incorrect actions. For instance, an AI providing incorrect medical advice or financial information falls into this category.
Two Axes of Hallucinations
To better understand hallucinations, we can consider two axes. Justification - whether the AI had information indicating that its statement was true. And truthfulness - whether the statement was actually true.

Based on these axes, we can classify hallucinations into four categories:
- Justified and True: The ideal scenario where the AI’s response is both correct and based on available information.
- Justified but False: The AI’s response is based on outdated or incorrect information. This can be fixed by improving the information available. For example, if an API response is ambiguous, it can be updated so that it is clear what it is referring to.
- Unjustified but True: The AI’s response is correct but not based on the information it was given. For example, the AI telling the customer that they should arrive at an airport 2 hours before a flight departure. If this was not grounded in, say, a knowledge base article, then this information is technically a hallucination even if it is true.
- Unjustified and False: The worst-case scenario where the AI’s response is both incorrect and not based on any available information. These are harmful hallucinations that could require an organization to reach out to the customer to fix the mistake.
Why Do Hallucinations Occur?
Hallucinations in generative AI occur due to several reasons. Generative models are inherently stochastic, meaning they can produce different outputs for the same input. Additionally, the large output space of these models increases the likelihood of errors, as they are capable of generating a wide range of responses. AI systems that rely on incomplete or outdated data are also prone to making incorrect statements. Finally, the complexity of instructions can result in misinterpretation, which may cause the model to generate unjustified responses.
Hallucination prevention and management
We typically think about four pillars when it comes to preventing and managing hallucinations:
- Preventing hallucinations from occurring in the first place
- Catching hallucinations before they reach the customer
- Tracking hallucinations post-production
- Improving the system
1. Preventing Hallucinations: Ensuring Responses are Properly Grounded
One of the most effective ways to prevent hallucinations is to ensure that AI responses are grounded in reliable data. This can be achieved through:
- Explicit Instructions: Providing clear and unambiguous instructions can help the AI interpret and respond accurately.
- API Responses: Integrating real-time data from APIs ensures that the AI has access to the most current information.
- Knowledge Base (KB) Articles: Relying on a well-maintained knowledge base can provide a solid foundation for AI responses.
2. Catching hallucinations: Approaches to Catch Hallucinations in Production
To minimize the risk of hallucinations, several safety mechanisms can be implemented:
- Input Safety Mechanisms: Detecting and filtering out abusive or out-of-scope requests before they reach the AI system can prevent inappropriate responses.
- Output Safety Mechanisms: Assessing the proposed AI response for safety and accuracy before it is delivered to the user. This can involve:some text
- Deterministic Checks using rules-based systems to ensure that certain types of language or content are never sent to users, or
- Classification Models, which employ machine learning models to classify and filter out potentially harmful responses. For example, a model can classify whether the information contained in a proposed response has been grounded on information retrieved from the right data sources. If this model suspects that the information is not grounded, it can reprompt the AI system to try again with this feedback.
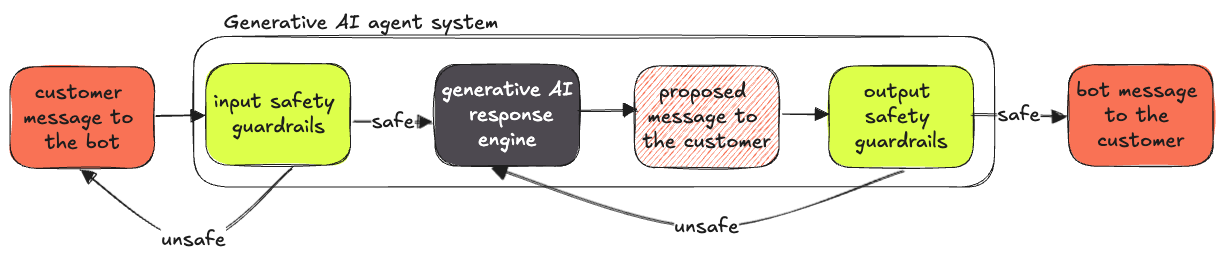
3. Tracking hallucinations: post-production
A separate post-production model can be used to classify AI responses as mistakes in more detail. While the “catching hallucination” model should balance effectiveness with latency, the post-production mistake monitoring model can be much larger, as latency is not a concern.
A well-defined hallucination taxonomy is crucial for systematically identifying, categorizing, and addressing errors in Generative AI systems. By having a well-defined error taxonomy system, users can aggregate reports that make it easy to identify, prioritize, and resolve issues quickly.
The following categories help identify the type of error, its source of misinformation, and the impact.
- Error Category -broad classification of types of the generative AI system errors.
- Error Type - specific nature or cause of an AI system error.
- Information Source - origin of data used by the AI system.
- System Source - component responsible for generating or processing AI output.
- Customer Impact Severity - level of negative effect on the customer.
4. Improving the system
Continuous improvement is crucial for managing and reducing hallucinations in AI systems. This involves several key practices. Regular updates ensure that the AI system is regularly updated with the latest data and information. Implementing feedback loops allows for the reporting and analysis of errors, which helps improve the system over time. Regular training and retraining of the AI model are essential to enable it to adapt to new data and scenarios. Finally, human oversight involving contact center supervisors to review and correct AI responses, especially in high-stakes situations, is critical.
Conclusion
By understanding the nature of hallucinations and implementing robust mechanisms to prevent, catch, and manage them, organizations can harness the power of Generative AI while minimizing risks. Just as human agents in contact centers are managed and coached to improve performance, Generative AI systems can also be continually refined to ensure they deliver accurate and reliable responses. By focusing on grounding responses in reliable data, employing safety mechanisms, and fostering continuous improvement, we can ensure that AI responses are safely grounded and free from harmful hallucinations.