Why your care strategy must consider issue complexity and urgency
A common trait of people working in technology is a desire to be able to cleanly categorize information, data, issues, etc. To be able to delineate between one bucket and another. We see this manifest in how companies think about customer conversations—should a conversation be automated? Yes or no? Does the customer need a live engagement for the entirety of the conversation vs. more asynchronous? Yes or no? But customer conversations aren’t actually so clearcut, and the needs don’t stay consistent as conversations and customer journeys go on.
At ASAPP, we have developed a fairly unique way of thinking about conversations. Rather than relying on a single intent to determine how the entire conversation should be handled, let’s look at each turn of the conversation to better inform what the next step should be. Every request has different needs, which change considerably based on various factors.
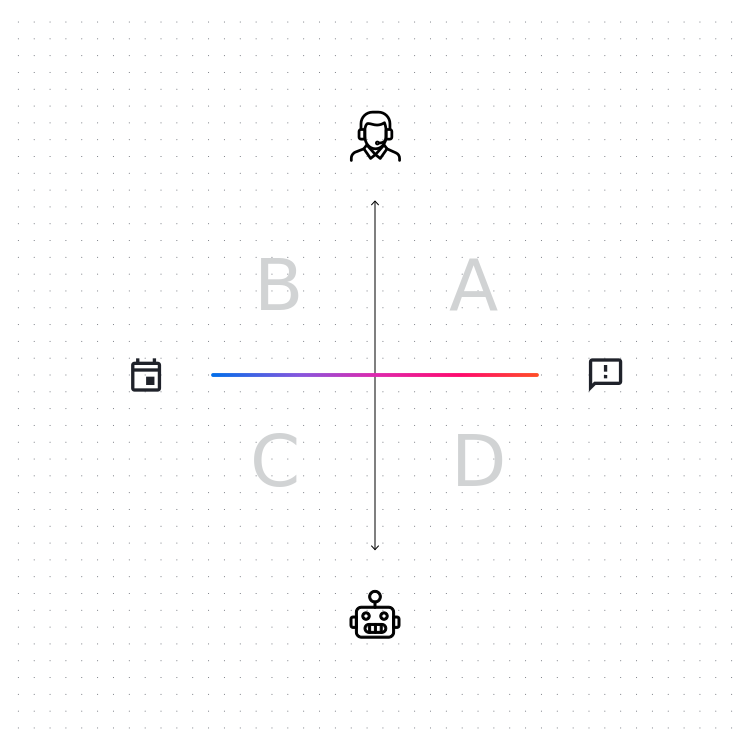
The above graph provides a nice illustration of how we can think about the issues. Along the y-axis, you have more complex vs. more simple interactions. At the bottom, you have conversations that are well served to be fully automated without any agent intervention. On the top, you have the opposite—conversations that benefit from having a skilled agent along for the ride. But those are extremes, most conversations fall in between the two, they require some human involvement and a bunch of automation. By thinking in very binary terms, automated or not automated, you lose out on all of the opportunities to reduce agent workload on a conversation by 20%, by 50%, by 75%. By treating each piece of a conversation as worthy of its own classification and diagnosis, you bring a lot of efficiency back into your business without risking frustrating your customer.
Now the x-axis, here we’re thinking about how routine vs. how urgent the issue is. It’s easy to think “we can serve customers asynchronously, they send an SMS. We get back to them when we get back to them, just like customers are used to interacting with friends and family.” But that leaves out a very important part of the picture. While many conversations are routine and can benefit from more asynchronous interactions, allowing companies to load balance workload on agents, there are cases where customers need urgent help—make a change to a flight about to take off, help resolve billing issue just before superbowl kick off, and in those cases, you don’t want to risk a customer not getting a response in time, especially not when so many conversions didn’t need that live resolution. Then there are cases just as with complexity vs. simplicity that are in between—an initial response might need help from a live agent, cutting off access to a bank account in the case of fraud, but the follow-ups and resolutions are well-served for asynchronous communication.

Customer interactions require different levels of attention. From simple routine issues to urgent complex requests, organizations must be able to seamlessly support every type of need, in the most efficient way possible, using the right mix of agent and automation.
Rachel Knaster
In addition to the content of what the customer is asking about, it’s important to take in every parameter you know about them and the context surrounding their issue. This goes far beyond simple intent classification. In order to determine the type of service customers need, you need to look at the entire weight of their requests. The best way to think about it is along axes of complexity and urgency.
Based on where they fall on this graph, customer interactions require different levels of attention. From simple routine issues (C) to urgent complex requests (A), organizations must be able to seamlessly support every type of need, in the most efficient way possible, using the right mix of agent and automation.
Is the customer’s question simple to solve? Then let’s automate it.
Is it complicated? Then let’s connect them with our frontline and have those agents do what they do best.
Is the issue one that can wait for an answer and more asynchronous by nature? Then let’s treat it that way.
Or is a customer’s flight about to take off and they need help? Let’s immediately connect them with someone.
These are fundamental questions contact centers should consider with every incoming request. There’s “no one size fits all” when it comes to CX strategy. Every interaction requires a different approach. so you can maximize throughput while keeping each customer satisfied.
Consider the graph above. Each quadrant represents a different category of request with its own unique considerations. In each case, the right mixture of live agent and AI, synchronous and asynchronous support can help solve the issue in the most optimal way possible. Here’s the ideal for each:
- Complex, urgent
- Agent-based, synchronous
- Low agent concurrency
- Automate part of agent workload
- Opportunity to mix voice and digital in same live conversation for faster resolution
- Complex, routine
- Agent-based, asynchronous
- Automate part of agent workload
- High agent concurrency
- Handoff to phone if required
- Simple, routine
- Fully automated interaction
- Low cost to serve
- Simple, urgent
- Fully automated, with fast escalation to live agent
- Complete history (context) of interaction required for agent
- Medium-high agent concurrency
- Automate part of agent workload
- Opportunity to mix voice and digital in same live conversation for faster resolution
While companies might prefer everything be automated or self service, that’s not always the most efficient way to solve an issue. Of course, neither is having your agents occupied addressing routine tasks all day. What’s needed is the right balance between the two—AI enhancing human performance so agents can handle more tasks and fully concentrate on those that need it. This is where more sophisticated machine learning offers incredible value.
There is an opportunity for AI to assist in every interaction, whether it’s handling the entire request or just part of the workload. While typically considered most helpful for automating simple tasks, the right AI models will improve over time, learning from customer interactions to assist with increasingly complex issues.
A single conversation can also become more simple or complex as it evolves, calling for changing levels of agent attention. For instance, now that the primary issue has been resolved, can the rest of this interaction be automated? Or has the issue escalated from automation to the need for an agent? Instant intent analysis provided by machine learning can help identify these occurrences to further optimize agent concurrency.
The truth is, sometimes the best thing is to have an agent live with just one customer, and sometimes it’s to have them handling multiple conversations. What’s important is for each organization to recognize the nuance and to build flexible solutions that adapt for the best outcomes to ensure operational performance is being enhanced, while never compromising on a personalized and connected experience for customers.